diff options
author | CoprDistGit <infra@openeuler.org> | 2023-06-20 05:47:21 +0000 |
---|---|---|
committer | CoprDistGit <infra@openeuler.org> | 2023-06-20 05:47:21 +0000 |
commit | 1c2d19d2214ce1cb5428bc82a4d25830a166ffb8 (patch) | |
tree | 8f56415a8024c9e94ee0c6542c668304280451e3 | |
parent | 19f7d4aff87c18da5309bffb45ed994c38e96616 (diff) |
automatic import of python-cdxbasicsopeneuler20.03
-rw-r--r-- | .gitignore | 1 | ||||
-rw-r--r-- | python-cdxbasics.spec | 2567 | ||||
-rw-r--r-- | sources | 1 |
3 files changed, 2569 insertions, 0 deletions
@@ -0,0 +1 @@ +/cdxbasics-0.2.58.tar.gz diff --git a/python-cdxbasics.spec b/python-cdxbasics.spec new file mode 100644 index 0000000..8f0e3d8 --- /dev/null +++ b/python-cdxbasics.spec @@ -0,0 +1,2567 @@ +%global _empty_manifest_terminate_build 0 +Name: python-cdxbasics +Version: 0.2.58 +Release: 1 +Summary: Basic Python tools +License: MIT License +URL: https://github.com/hansbuehler/cdxbasics +Source0: https://mirrors.aliyun.com/pypi/web/packages/a7/fa/e51bf12b0673056a82340cbf98d323d7843a427d59d5738c5ef9e9a01913/cdxbasics-0.2.58.tar.gz +BuildArch: noarch + +Requires: python3-numpy +Requires: python3-pandas +Requires: python3-matplotlib +Requires: python3-sortedcontainers +Requires: python3-psutil + +%description +# cdxbasics
+
+Collection of basic tools for Python development.
+
+Install by
+
+ conda install cdxbasics -c hansbuehler
+
+or
+
+ pip install cdxbasics
+
+## dynaplot
+
+Tools for dynamic (animated) plotting in Jupyer/IPython. The aim of the toolkit is making it easy to develop visualization with `matplotlib` which dynamically updates, for example during training with machine learing kits such as `tensorflow`. This has been tested with Anaconda's JupyterHub and `%matplotlib inline`.
+
+Some users reported that the package does not work in some versions of Jupyter. In this case, please try setting `dynaplot.DynamicFig.MODE = 'canvas'`. I appreciate if you let me know whether this resolved
+the problem.
+
+#### Animated Matplotlib in Jupyter
+
+See the jupyter notebook [notebooks/DynamicPlot.ipynb](https://github.com/hansbuehler/cdxbasics/blob/master/cdxbasics/notebooks/DynamicPlot.ipynb) for some applications.
+
+
+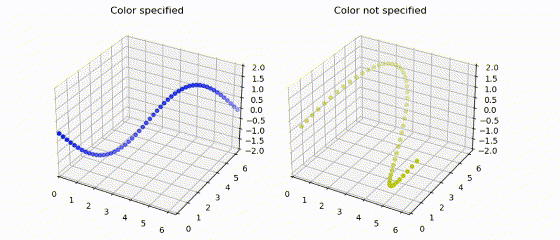
+
+ # example
+ %matplotlib inline
+ import numpy as np
+ x = np.linspace(-5,5,21)
+ y = np.ramdom.normal(size=(21,5))
+
+ # create figure
+ from cdxbasics.dynaplot import figure
+ fig = figure() # equivalent to matplotlib.figure
+ ax = fig.add_subplot() # no need to specify row,col,num
+ l = ax.plot( x, y[:,0] )[0] # get fist line2D object
+ fig.render() # construct figure & draw graph
+
+ # animate
+ import time
+ for i in range(1,5):
+ time.sleep(1)
+ l.set_ydata( y[:,i] ) # update data
+ fig.render()
+
+ fig.close() # clear figure to avoid duplication
+
+See example notebook for how to use the package for lines, confidence intervals, and 3D graphs.
+
+#### Simpler sub_plot
+
+The package lets you create sub plots without having to know the number of plots in advance: you do not need to specify `rol, col, num` when calling `add_subplot`. The underlying figure object will automatically arrange them on a grid for you.
+
+ # create figure
+ from cdxbasics.dynaplot import figure
+ fig = figure(col_size=4, row_size=4, col_num=3)
+ # equivalent to matplotlib.figure
+ ax = fig.add_subplot() # no need to specify row,col,num
+ ax.plot( x, y )
+ ax = fig.add_subplot() # no need to specify row,col,num
+ ax.plot( x, y )
+ ...
+ fig.next_row() # another row
+ ax = fig.add_subplot() # no need to specify row,col,num
+ ax.plot( x, y )
+ ...
+
+ fig.render() # draws the plots
+
+#### Other features
+
+
+
+There are a number of other functions to aid plotting
+
+* `figure()` which returns a `DynamicFig` object:
+
+ Function to replace `matplotlib.figure` which will defer creation of the figure until the first call of `render()`. The effect is that we no longer need to provide the total number of rows and columns in advance - i.e. you won't need to call the equivalent of `fig.add_subplot(3,4,14)` but can just call `fig.add_subplot()`.
+
+ * Instead of `figsize` the function `figure()` accepts `row_size`, `col_size` and `col_nums` to dynamically generate an appropriate figure size.
+
+ Key member functions of `DynamicFig` are:
+ * `add_subplot` to add a new plot. No arguments needed.
+ * `next_row()` to skip to the next row.
+ * `render()` to draw the figure. When called the first time will create all the underlying matplotlib objects. Subsequent calls will re-draw the canvas if the figure was modified. See examples in https://github.com/hansbuehler/cdxbasics/blob/master/cdxbasics/notebooks/DynamicPlot.ipynb
+ * `close()` to close the figure. If not called, Jupyter creates an unseemly second copy of the graph when the current cell is finished running.
+
+* `color_css4, color_base, color_tableau, color_xkcd`:
+
+ Each function returns the $i$th element of the respective matplotlib color
+ table. The purpose is to simplify using consistent colors accross different plots.
+
+ Example:
+
+ fig = dynaplot.figure()
+ ax = fig.add_subplot()
+ # draw 10 lines in the first sub plot, and add a legend
+ for i in range(10):
+ ax.plot( x, y[i], color=color_css4(i), label=labels[i] )
+ ax.legend()
+ # draw 10 lines in the second sub plot. No legend needed as colors are shared with first plot
+ ax = fig.add_subplot()
+ for i in range(10):
+ ax.plot( x, z[i], color=color_css4(i) )
+ fig.render()
+
+* `colors_css4, colors_base, colors_tableau, colors_xkcd`:
+
+ Generator versions of the `color_` functions.
+
+## prettydict
+
+A number of simple extensions to standard dictionaries which allow accessing any element of the dictionary with "." notation. The purpose is to create a functional-programming style method of generating complex objects.
+
+ from cdxbasics.prettydict import PrettyDict
+ pdct = PrettyDict(z=1)
+ pdct['a'] = 1 # standard dictionary write access
+ pdct.b = 2 # pretty write access
+ _ = pdct.b # read access
+ _ = pdct("c",3) # short cut for pdct.get("c",3)
+
+There are three versions:
+
+* `PrettyDict`:
+ Pretty version of standard dictionary.
+* `PrettyOrderedDict`:
+ Pretty version of ordered dictionary.
+* `PrettySortedDict`:
+ Pretty version of sorted dictionary.
+
+#### Assigning member functions
+
+"Pretty" objects also allow assigning bona fide member functions by a simple semantic of the form:
+
+ def mult_b( self, x ):
+ return self.b * x
+ pdct = mult_a
+
+Calling `pdct.mult_a(3)` with above config will return `6` as expected. This only works when using the member synthax for assigning values
+to a pretty dictionary; if the standard `[]` operator is used then functions will be assigned to the dictionary as usual, hence they are static members of the object.
+
+The reason for this is as follows: consider
+
+ def mult( a, b ):
+ return a*b
+ pdct.mult = mult
+ mult(3,4) --> produces am error as three arguments as are passed if we count 'self'
+
+ In this case, use:
+
+ pdct['mult'] = mult
+ pdct.mult(3,4) --> 12
+
+
+## config
+
+Tooling for setting up program-wide configuration. Aimed at machine learning programs to ensure consistency of code accross experimentation.
+
+ from cdxbasics.config import Config
+ config = Config()
+
+**Key features**
+
+* Detect misspelled parameters by checking that all parameters of a config have been read.
+* Provide summary of all values read, including summary help for what they were for.
+* Nicer synthax than dictionary notation, in particular for nested configurations.
+* Simple validation to ensure values are within a given range or from a list of options.
+
+#### Creating configs
+
+Set data with both dictionary and member notation:
+
+ config = Config()
+ config['features'] = [ 'time', 'spot' ]
+ config.weights = [ 1, 2, 3 ]
+
+Create sub configurations with member notation
+
+ config.network.depth = 10
+ config.network.activation = 'relu'
+ config.network.width = 100
+
+This is equivalent to
+
+ config.network = Config()
+ config.network.depth = 10
+ config.network.activation = 'relu'
+ config.network.width = 100
+
+#### Reading a config
+
+When reading the value of a `key` from config, `config.__call__()` uses a default value, and a cast type. It first attempts to find `key` in the `config`.
+* If `key` is found, it casts the value provided for `key` using the `cast` type and returned.
+* If `key` is not found, then the default value will be cast using `cast` type and returned.
+
+The function also takes a `help` text which allows providing live information on what variable are read from the config. The latter is used by the function `usage_report()` which therefore provides live documentation of the code which uses the config object.
+
+ class Network(object):
+ def __init__( self, config ):
+ # read top level parameters
+ self.features = config("features", [], list, "Features for the agent" )
+ self.weights = config("weights", [], np.asarray, "Weigths for the agent", help_default="no initial weights")
+ config.done() # see below
+
+In above example any data provided for they keywords `weigths` will be cast using `numpy.asarray`.
+
+Further parameters of `()` are the help text, plus ability to provide text versions of the default with `help_default` (e.g. if the default value is complex), and the cast operator with `help_cast` (again if the
+respective operation is complex).
+
+__Important__: the `()` operator does not have a default value unless specified. If no default value is specified, and the key is not found, then a KeyError is generated.
+
+You can read sub-configurations with the previsouly introduced member notation:
+
+ self.activation = config.network("activation", "relu", str, "Activation function for the network")
+
+An alternative is the explicit:
+
+ network = config.network
+ self.depth = network('depth', 10000, int, "Depth for the network")
+
+#### Imposing simple restrictions on values
+
+We can impose simple restrictions to any values read from a config. To this end, import the respective type operators:
+
+ from cdxbasics.config import Int, Float
+
+One-sided restriction:
+
+ # example enforcing simple conditions
+ self.width = network('width', 100, Int>3, "Width for the network")
+
+Restrictions on both sides of a scalar:
+
+ # example encorcing two-sided conditions
+ self.percentage = network('percentage', 0.5, ( Float >= 0. ) & ( Float <= 1.), "A percentage")
+
+Enforce the value being a member of a list:
+
+ # example ensuring a returned type is from a list
+ self.ntype = network('ntype', 'fastforward', ['fastforward','recurrent','lstm'], "Type of network")
+
+We can allow a returned value to be one of several casting types by using tuples. The most common use case is that `None` is a valid value for a config, too. For example, assume that the `name` of the network model should be a string or `None`. This is implemented as
+
+ # example allowing either None or a string
+ self.keras_name = network('name', None, (None, str), "Keras name of the network model")
+
+We can combine conditional expressions with the tuple notation:
+
+ # example allowing either None or a positive int
+ self.batch_size = network('batch_size', None, (None, Int>0), "Batch size or None for TensorFlow's default 32", help_cast="Positive integer, or None")
+
+
+#### Ensuring that we had no typos & that all provided data is meaningful
+
+A common issue when using dictionary-based code is that we might misspell one of the parameters. Unless this is a mandatory parameter we might not notice that we have not actually changed its value in the code below.
+
+To check that all values of `config` are read use `done()`
+
+ config.done() # checks that we have read all keywords.
+
+It will alert you if there are keywords or children which haven't been read. Most likely, those will be typos. Consider the following example where `width` is misspelled in our config:
+
+ class Network(object):
+ def __init__( self, config ):
+ # read top level parameters
+ self.depth = config("depth", 1, Int>=1, "Depth of the network")
+ self.width = config("width", 3, Int>=1, "Width of the network")
+ self.activaton = config("activation", "relu", help="Activation function", help_cast="String with the function name, or function")
+ config.done() # <-- test that all members of config where read
+
+ config = Config()
+ config.features = ['time', 'spot']
+ config.network.depth = 10
+ config.network.activation = 'relu'
+ config.network.widht = 100 # (intentional typo)
+
+ n = Network(config.network)
+
+Since `width` was misspelled in setting up the config, you will get a warning to this end:
+
+ Error closing 'config.network': the following config arguments were not read: ['widht']
+
+ Summary of all variables read from this object:
+ config.network['activation'] = relu # Activation function; default: relu
+ config.network['depth'] = 10 # Depth of the network; default: 1
+ config.network['width'] = 3 # Width of the network; default: 3
+
+Note that you can also call `done()` at top level:
+
+ class Network(object):
+ def __init__( self, config ):
+ # read top level parameters
+ self.depth = config("depth", 1, Int>=1, "Depth of the network")
+ self.width = config("width", 3, Int>=1, "Width of the network")
+ self.activaton = config("activation", "relu", help="Activation function", help_cast="String with the function name, or function")
+
+ config = Config()
+ config.features = ['time', 'spot']
+ config.network.depth = 10
+ config.network.activation = 'relu'
+ config.network.widht = 100 # (intentional typo)
+
+ n = Network(config.network)
+ test_features = config("features", [], list, "Features for my network")
+ config.done()
+
+produces
+
+ ERROR:x:Error closing 'config.network': the following config arguments were not read: ['widht']
+
+ Summary of all variables read from this object:
+ config.network['activation'] = relu # Activation function; default: relu
+ config.network['depth'] = 10 # Depth of the network; default: 1
+ config.network['width'] = 3 # Width of the network; default: 3
+ #
+ config['features'] = ['time', 'spot'] # Default: 2
+
+You can check the status of the use of the config by using the `not_done` property.
+
+
+#### Detaching child configs and other Copy operations
+
+You can also detach a child config, which allows you to store it for later use without triggering `done()` errors:
+
+ def read_config( self, confg ):
+ ...
+ self.config_training = config.training.detach()
+ config.done()
+
+`detach()` will mark he original child as 'done'. Therefore, we will need to call `done()` again, when we finished processing the detached child:
+
+ def training(self)
+ epochs = self.config_training("epochs", 100, int, "Epochs for training")
+ batch_size = self.config_training("batch_size", None, help="Batch size. Use None for default of 32" )
+
+ self.config_training.done()
+
+Use `copy()` to make a bona fide copy of a child, without marking the source child as 'done'. `copy()` will return a config which shares the same status as the source object. If you want an "unused" copy, use `clean_copy()`. A virtual clone is created via `clone()`. A cloned config stores information on usage in the same place for the original object. This is also the semantic of the copy constructor.
+
+#### Self-recording all available configuration parameters
+
+Once your program ran, you can read the summary of all values, their defaults, and their help texts.
+
+ print( config.usage_report( with_cast=True ) )
+
+Prints:
+
+ config.network['activation'] = relu # (str) Activation function for the network; default: relu
+ config.network['depth'] = 10 # (int) Depth for the network; default: 10000
+ config.network['width'] = 100 # (int>3) Width for the network; default: 100
+ config.network['percentage'] = 0.5 # (float>=0. and float<=1.) Width for the network; default: 0.5
+ config.network['ntype'] = 'fastforward' # (['fastforward','recurrent','lstm']) Type of network; default 'fastforward'
+ config.training['batch_size'] = None # () Batch size. Use None for default of 32; default: None
+ config.training['epochs'] = 100 # (int) Epochs for training; default: 100
+ config['features'] = ['time', 'spot'] # (list) Features for the agent; default: []
+ config['weights'] = [1 2 3] # (asarray) Weigths for the agent; default: no initial weights
+
+#### Calling functions with named parameters:
+
+ def create_network( depth=20, activation="relu", width=4 ):
+ ...
+
+We may use
+
+ create_network( **config.network )
+
+However, there is no magic - this function will mark all direct members (not children) as 'done' and will not record the default values of the function `create_network`. Therefore `usage_report` will be somewhat useless. This method will still catch unused variables as "unexpected keyword arguments".
+
+#### Unique ID
+
+Another common use case is that we wish to cache some process in a complex operation. Assuming that the `config` describes all relevant parameters
+we can use `config.unique_id()` to obtain a unique hash ID for the given config.
+
+This can be used, for example, as file name for caching. See also `cdxbasics.subdir` below.
+
+#### Advanced **kwargs Handling
+
+The `Config` class can be used to improve `kwargs` handling.
+Assume we have
+
+ def f(**kwargs):
+ a = kwargs.get("difficult_name", 10)
+ b = kwargs.get("b", 20)
+
+We run the usual risk of somebody mispronouncing the parameter name which we would never know. Therefore we may improve upon the above with
+
+ def f(**kwargs):
+ kwargs = Config(kwargs)
+ a = kwargs("difficult_name", 10)
+ b = kwargs("b", 20)
+ kwargs.done()
+
+If now a user calls `f` with a misspelled `config(difficlt_name=5)` an error will be raised.
+
+Another pattern is to allow both `config` and `kwargs`:
+
+ def f( config=Config(), **kwargs):
+ kwargs = config.detach.update(kwargs)
+ a = kwargs("difficult_name", 10)
+ b = kwargs("b", 20)
+ kwargs.done()
+
+## logger
+
+Tools for defensive programming a'la the C++ ASSERT/VERIFY macros. Aim is to provide one line validation of inputs to functions with intelligible error messages:
+
+ from cdxbasics.logger import Logger
+ _log = Logger(__file__)
+ ...
+ def some_function( a, ...):
+ _log.verify( a==1, "'a' is not one but %s", a)
+ _log.warn_if( a!=1, "'a' was not one but %s", a)
+
+#### Member functions; mostly self-explanatory:
+
+Exceptions independent of logging level
+
+ verify( cond, text, *args, **kwargs )
+ If cond is not met, raise an exception with util.fmt( text, *args, **kwargs ). This is the Python version of C++ VERIFY
+
+ throw_if(cond, text, *args, **kwargs )
+ If cond is met, raise an exception with util.fmt( text, *args, **kwargs )
+
+ throw( text, *args, **kwargs )
+ Just throw an exception with util.fmt( text, *args, **kwargs )
+
+Unconditional logging
+
+ debug( text, *args, **kwargs )
+ info( text, *args, **kwargs )
+ warning( text, *args, **kwargs )
+ error( text, *args, **kwargs )
+ critical( text, *args, **kwargs )
+
+ throw( text, *args, **kwargs )
+
+Verify-conditional functions
+
+ # raise an exception if 'cond' is not True
+ verify( cond, text, *args, **kwargs )
+
+ # print log message of respective level if 'cond' is not True
+ verify_debug( cond, text, *args, **kwargs )
+ verify_info( cond, text, *args, **kwargs )
+ verify_warning( cond, text, *args, **kwargs )
+
+If-conditional functions
+
+ # raise an exception if 'cond' is True
+ throw_if( cond, text, *args, **kwargs )
+
+ # write log message if 'cond' is True
+ debug_if( cond, text, *args, **kwargs )
+ info_if( cond, text, *args, **kwargs )
+ warning_if( cond, text, *args, **kwargs )
+
+ # print message if 'cond' is True
+ prnt_if( cond, text, *args, **kwargs ) # with EOL
+ write_if( cond, text, *args, **kwargs ) # without EOL
+
+## subdir
+
+A few tools to handle file i/o in a transparent way, focusing on caching data. The key idea is to provide transparent, concise pickle access to the file system in a manner similar to dictionary access. Files managed by `subdir` also all have the same extension, which is `pck` by default.
+
+#### Key pattern:
+
+Our pattern assumes that each calcuation is determined by a number of parameters for which we can compute a unique (file) ID for caching results. Unique file IDs can be computed using `uniqueFileName48()`. Here is an example which assumes that `None` is not a valid return value for the underlying function code:
+
+
+ from cdxbasics.config import Config
+ from cdxbasics.subdir import SubDir, CacheMode, uniqueFileName48
+
+ def function_with_caching( config ):
+ # determine caching strategy
+ cache_mode = config.caching("mode", CacheMode.ON, CacheMode.MODES, "Caching strategy: " + CacheMode.HELP)
+ cache_dir = config.caching("directory", "caching", str, "Caching directory")
+ cache_id = config.function.unique_id(length=48)
+
+ # check whether we should delete any existing files
+ if cache_mode.delete:
+ cache_dir.delete(cache_id)
+
+ # read existing file, if desired and possible
+ data_of_my_function = cache_dir.read(cache_id) if cache_mode.read else None
+
+ # check whether we need to compute some data
+ if data_of_my_function is None:
+ ....
+ data_of_my_function = .... use config.function for settings
+ ....
+
+ # write back to disk
+ if cache_node.write:
+ cache_dir.write(cache_id, data_of_my_function)
+
+ return data_of_my_function
+
+See also the example for `CacheMode` below.
+
+
+#### Creating directories
+
+You can create directories using the `SubDir` class. Simply write
+
+ subdir = SubDir("my_directory") # relative to current working directory
+ subdir = SubDir("./my_directory") # relative to current working directory
+ subdir = SubDir("~/my_directory") # relative to home directory
+ subdir = SubDir("!/my_directory") # relative to default temp directory
+
+You can specify a parent for relative path names:
+
+ subdir = SubDir("my_directory", "~") # relative to home directory
+
+Change the extension to `bin`
+
+ subdir = SubDir("~/my_directory;*.bin")
+ subdir = SubDir("~/my_directory", ext="bin")
+ subdir = SubDir("my_directory", "~", ext="bin")
+
+You can also use the `()` operator to generate sub directories. This operator is overloaded: for a single argument, it creates a relative sub-directory:
+
+ parent = SubDir("~/parent")
+ subdir = parent("subdir")
+
+Be aware that when the operator `()` is called with two arguments, then it reads files; see below.
+
+You can obtain a list of all sub directories in a directory by using `subDirs()`.
+
+#### I/O
+##### Reading
+
+To read the data contained in a file 'file.pck' in our subdirectory with extension 'pck' use either of the following
+
+ data = subdir.read("file") # returns the default if file is not found
+ data = subdir.read("file", default=None) # returns the default if file is not found
+
+This function will return `None` by default if 'file' does not exist. You can make it throw an error by calling `subdir.read("file", throwOnError=True)` instead.
+
+You can also use the `()` operator, in which case you must specify a default value (if you don't, then the operator will return a sub directory):
+
+ data = subdir("file", None) # returns None if file is not found
+
+You can also use both member and item notation to access files. In this case, though, an error will be thrown if the file does not exist
+
+ data = subdir.file # raises AtttributeError if file is not found
+ data = subdir['file'] # raises KeyError if file is not found
+
+You can read a range of files in one function call:
+
+ data = subdir.read( ["file1", "file2"] )
+
+Finally, you can also iterate through all existing files:
+
+ for file in subdir:
+ data = subdir.read(file)
+ ...
+
+To obtain a list of all files in our directory which have the correct extension, use `keys()`.
+
+##### Writing
+
+To write data, use any of
+
+ subdir.write("file", data)
+ subdir.file = data
+ subdir['file'] = data
+
+To write several files at once, write
+
+ subdir.write(["file1", "file"], [data1, data2])
+
+Note that when writing to an object, `subdir` will first write to a temporary file, and then rename this file into the target file name. The temporary file name is a `util.uniqueHash48` generated from the target file name, current time, process and thread ID, as well as the machines's UUID. This is done to reduce collisions between processes/machines accessing the same files. It does not remove collision risk entirely, though.
+
+##### Test existence of files
+
+To test existence of 'file' in a directory, use one of
+
+ subdir.exist('file')
+ 'file' in subdir
+
+#### Deleting files
+
+To delete a 'file', use any of the following:
+
+ subdir.delete(file)
+ del subdir.file
+ del subdir['file']
+
+All of these are _silent_, and will not throw errors if 'file' does not exist. In order to throw an error use
+
+ subdir.delete(file, raiseOnError=True)
+
+Other file and directoru deletion methods:
+
+* `deleteAllKeys`: delete all files in the directory, but do not delete sub directories or files with extensions different to our own.
+* `deleteAllContent`: delete all files with our extension, and all sub directories.
+* `eraseEverything`: delete everything
+
+## util
+
+A collection of utility functions.
+
+### uniqueHash
+
+ uniqueHash( *kargs, **kwargs )
+ uniqueHash32( *kargs, **kwargs )
+ uniqueHash48( *kargs, **kwargs )
+ uniqueHash64( *kargs, **kwargs )
+
+Each of these functions returns a unique hash key for the arguments provided for the respective function. The functions *32,*48,*64 return hashes of the respective length, while `uniqueHash` returns the hashes of standard length. These functions will make an effort to robustify the hashes against Python particulars: for example, dictionaries are hashed with sorted keys.
+
+**These functions will ignore all dictionary or object members starting with "`_`".** They also will by default not hash _functions_ or _properties_.
+This is sometimes undesitable, for example when functions are configuration elements:
+
+ config = Config()
+ config.f = lambda x : x**2
+
+
+
+To change this behavuour, use `uniqueHashExt( length : int, parse_functions : bool = False, parse_underscore : str = "nonee")` which returns a hash function of desired lengths with the option to parse elements starting with "`_`" as well.
+
+### CacheMode
+
+A simple enum-type class to help implement a standard caching pattern.
+It implements the following decision matrix
+
+| |on |gen |off |update |clear |readonly|
+|----------------------------------------|------|------|--------|---------|--------|--------|
+|load cache from disk if exists |x |x |- |- |- |x|
+|write updates to disk |x |x |- |x |- |-|
+|delete existing object |- |- |- |- |x |-|
+|delete existing object if incompatible |x |- |- |x |x |-|
+
+Typically, the user is allowed to set the desired `CacheMode` using a `Config` element. The corresponding `CacheMode` object then implements the properties `read`, `write`, `delete` and `del_incomp`.
+
+Prototype code is to be implemented as follows:
+
+ def compute_cached( ..., cache_mode, cache_dir ):
+
+ unique_id = unqiueHash48( ... ) # compute a unique hash for the object
+
+ # delete existing cache if requested
+ if cache_mode.delete:
+ cache_dir.delete(unique_id)
+
+ # attempt to read cache
+ ret = cache_dir.read(unique_id) if cache_mode.read else None
+
+ # validate cache, e.g. is it of the right version
+ if not ret is None:
+ # validate that 'ret is a valid object
+ if not is_valid(ret):
+ if cache_model.del_incomp:
+ cache_dir.delete(unqiue_id)
+ ret = None
+
+ # compute new object if need be
+ if ret is None:
+ # compute new object
+ ret = ...
+
+ # write new object to disk
+ if cache_mode.write:
+ cache_dir.write(unique_id, ret)
+
+ return ret
+
+### WriteLine
+
+A simple utility class to manage printing in a given line with carriage returns (`\r`).
+Essentially, it keeps track of the length what was printed so far at the current line. If a `\r` is encountered it will clear the rest of the line to avoid having residual text from the previous line.
+
+Example 1 (how to use \r and \n)
+
+ write = WriteLine("Initializing...")
+ import time
+ for i in range(10):
+ time.sleep(1)
+ write("\rRunning %g%% ...", round(float(i+1)/float(10)*100,0))
+ write(" done.\nProcess finished.\n")
+
+Example 2 (line length is getting shorter)
+
+ write = WriteLine("Initializing...")
+ import time
+ for i in range(10):
+ time.sleep(1)
+ write("\r" + ("#" * (9-i)))
+ write("\rProcess finished.\n")
+
+### Misc
+
+* `fmt()`: C++ style format function.
+* `plain()`: converts most combinations of standards elements or objects into plain list/dict structures.
+* `isAtomic()`: whether something is string, float, int, bool or date.
+* `isFloat()`: whether something is a float, including a numpy float.
+* `isFunction()`: whether something is some function.
+* `bind()`: simple shortcut to bind function parameters, e.g.
+
+ def f(a, b, c):
+ pass
+ f_a = bind(f, a=1)
+
+* `fmt_list()` returns a nicely formatted list, e.g. `fmt_list([1,2,3])` returns `1, 2 and 3`.
+
+* `fmt_dict()` returns a nicely formatted dictrionary, e.g. `fmt_dict({'a':1,'b':'test'})` returns `a: 1, b: test`.
+* `fmt_seconds()` returns string for seconds, e.g. `fmt_seconds(10)` returns `10s` while `fmt_seconds(61)` returns `1:00`.
+* `fmt_big_number()` converts a large integer into an abbreviated string with terminating `K`, `M`, `B`, `T` as appropriate, using base 10.<br>
+ For example `fmt_big_number(12345)` returns `12.35K`.
+* `fmt_big_byte_number()` converts a large integer into an abbreviated string with terminating `K`, `M`, `G`, `T` as appropriate, here using base 16.<br>For example `fmt_big_byte_number(12345)` returns `12.06K`.
+* `fmt_datetime()` returns a nicely formatted daytime code in natural order e.g. YYYY-MM-DD HH:SS.<br>It returns the respective simplification if just a `date` or `time` is passed instead of a `datetime`.
+
+* `is_jupyter()` tries to assess whether the current environment is a jupyer IPython environment.
+This is experimental as it appears there is no safe way to do this. The current implemenentation checks whether the command which started the current process contains the string `jupyter`.
+
+## np
+
+A small number of statistical numpy functions which take a weight vector (distribution) into account, namely
+
+* `mean(P,x,axis)` computes the mean of `x` using the distribution `P`. If `P` is None, it returns `numpy.mean(x,axis)`.
+* `var(P,x,axis)` computes the variance of `x` using the distribution `P`. If `P` is None, it returns `numpy.var(x,axis)`.
+* `std(P,x,axis)` computes the standard deviation of `x` using the distribution `P`. If `P` is None, it returns `numpy.std(x,axis)`.
+* `err(P,x,axis)` computes the standard error of `x` using the distribution `P`. If `P` is None, it returns `numpy.std(x,axis)/sqrt(x.shape[axis])`.
+
+Two further functions are used to compute binned statistics:
+
+* `mean_bins(x,bins,axis,P)` computes the means of `x` over equidistant `bins` using the distribition `P`.
+* `mean_std_bins(x,bins,axis,P)` computes the means and standard deviations of `x` over equidistant `bins` using the distribition `P`.
+
+
+
+## verbose
+
+**The `verbose` interface has changed in 0.2.36**
+
+This module provides the `Context` utility class for printing 'verbose' information, with indentation depending on the detail level.
+
+The basic idea is that the root context has level 0, with increasing levels for sub-contexts. When printing information, we can (a) limit printing up to a given level and (b) automatically indent the output to reflect the current level of detail.
+
+* Create a `Context` model, and define its verbosity in its constructor, e.g. `all`, `none` or a number. A negative number means that no outout will be generated (`quiet`), while `None` means all output will be printed (`all`). Sub-contexts inherent verbosity from their parents.
+* To write a text at current level to `stdout` use `write()`.
+* To write a text at a sub-level use `report()`. You can also use the overloaded call operator.
+* To create a sub-context, either call `sub()` or use the overloaded call operator.
+
+Here is an example:
+
+ from cdxbasics.verbose import Context, quiet
+
+ def f_sub( num=10, context = quiet ):
+ context.report(0, "Entering loop")
+ for i in range(num):
+ context.report(1, "Number %ld", i)
+
+ def f_main( context = quiet ):
+ context.write( "First step" )
+ # ... do something
+ context.report( 1, "Intermediate step 1" )
+ context.report( 1, "Intermediate step 2\nwith newlines" )
+ # ... do something
+ f_sub( context=context(1) ) # call function f_sub with a sub-context
+ # ... do something
+ context.write( "Final step" )
+
+ print("Verbose=1")
+ context = Context(1)
+ f_main(context)
+
+ print("\nVerbose=2")
+ context = Context(2)
+ f_main(context)
+
+ print("\nVerbose='all'")
+ context = Context('all')
+ f_main(context)
+
+ print("\nVerbose='quiet'")
+ context = Context('quiet')
+ f_main(context)
+
+Returns
+
+ Verbose=1
+ 00: First step
+ 01: Intermediate step 1
+ 01: Intermediate step 2
+ 01: with newlines
+ 01: Entering loop
+ 00: Final step
+
+ Verbose=2
+ 00: First step
+ 01: Intermediate step 1
+ 01: Intermediate step 2
+ 01: with newlines
+ 01: Entering loop
+ 02: Number 0
+ 02: Number 1
+ 02: Number 2
+ 02: Number 3
+ 02: Number 4
+ 02: Number 5
+ 02: Number 6
+ 02: Number 7
+ 02: Number 8
+ 02: Number 9
+ 00: Final step
+
+ Verbose='all'
+ 00: First step
+ 01: Intermediate step 1
+ 01: Intermediate step 2
+ 01: with newlines
+ 01: Entering loop
+ 02: Number 0
+ 02: Number 1
+ 02: Number 2
+ 02: Number 3
+ 02: Number 4
+ 02: Number 5
+ 02: Number 6
+ 02: Number 7
+ 02: Number 8
+ 02: Number 9
+ 00: Final step
+
+ Verbose='quiet'
+
+The purpose of initializing functions usually with `quiet` is that they can be used accross different contexts without printing anything by default.
+
+ + +%package -n python3-cdxbasics +Summary: Basic Python tools +Provides: python-cdxbasics +BuildRequires: python3-devel +BuildRequires: python3-setuptools +BuildRequires: python3-pip +%description -n python3-cdxbasics +# cdxbasics
+
+Collection of basic tools for Python development.
+
+Install by
+
+ conda install cdxbasics -c hansbuehler
+
+or
+
+ pip install cdxbasics
+
+## dynaplot
+
+Tools for dynamic (animated) plotting in Jupyer/IPython. The aim of the toolkit is making it easy to develop visualization with `matplotlib` which dynamically updates, for example during training with machine learing kits such as `tensorflow`. This has been tested with Anaconda's JupyterHub and `%matplotlib inline`.
+
+Some users reported that the package does not work in some versions of Jupyter. In this case, please try setting `dynaplot.DynamicFig.MODE = 'canvas'`. I appreciate if you let me know whether this resolved
+the problem.
+
+#### Animated Matplotlib in Jupyter
+
+See the jupyter notebook [notebooks/DynamicPlot.ipynb](https://github.com/hansbuehler/cdxbasics/blob/master/cdxbasics/notebooks/DynamicPlot.ipynb) for some applications.
+
+
+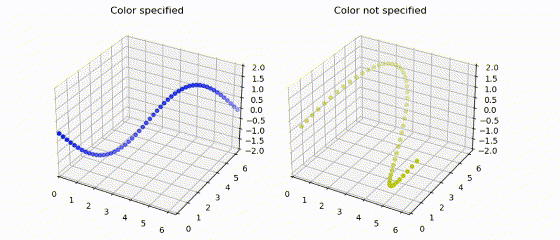
+
+ # example
+ %matplotlib inline
+ import numpy as np
+ x = np.linspace(-5,5,21)
+ y = np.ramdom.normal(size=(21,5))
+
+ # create figure
+ from cdxbasics.dynaplot import figure
+ fig = figure() # equivalent to matplotlib.figure
+ ax = fig.add_subplot() # no need to specify row,col,num
+ l = ax.plot( x, y[:,0] )[0] # get fist line2D object
+ fig.render() # construct figure & draw graph
+
+ # animate
+ import time
+ for i in range(1,5):
+ time.sleep(1)
+ l.set_ydata( y[:,i] ) # update data
+ fig.render()
+
+ fig.close() # clear figure to avoid duplication
+
+See example notebook for how to use the package for lines, confidence intervals, and 3D graphs.
+
+#### Simpler sub_plot
+
+The package lets you create sub plots without having to know the number of plots in advance: you do not need to specify `rol, col, num` when calling `add_subplot`. The underlying figure object will automatically arrange them on a grid for you.
+
+ # create figure
+ from cdxbasics.dynaplot import figure
+ fig = figure(col_size=4, row_size=4, col_num=3)
+ # equivalent to matplotlib.figure
+ ax = fig.add_subplot() # no need to specify row,col,num
+ ax.plot( x, y )
+ ax = fig.add_subplot() # no need to specify row,col,num
+ ax.plot( x, y )
+ ...
+ fig.next_row() # another row
+ ax = fig.add_subplot() # no need to specify row,col,num
+ ax.plot( x, y )
+ ...
+
+ fig.render() # draws the plots
+
+#### Other features
+
+
+
+There are a number of other functions to aid plotting
+
+* `figure()` which returns a `DynamicFig` object:
+
+ Function to replace `matplotlib.figure` which will defer creation of the figure until the first call of `render()`. The effect is that we no longer need to provide the total number of rows and columns in advance - i.e. you won't need to call the equivalent of `fig.add_subplot(3,4,14)` but can just call `fig.add_subplot()`.
+
+ * Instead of `figsize` the function `figure()` accepts `row_size`, `col_size` and `col_nums` to dynamically generate an appropriate figure size.
+
+ Key member functions of `DynamicFig` are:
+ * `add_subplot` to add a new plot. No arguments needed.
+ * `next_row()` to skip to the next row.
+ * `render()` to draw the figure. When called the first time will create all the underlying matplotlib objects. Subsequent calls will re-draw the canvas if the figure was modified. See examples in https://github.com/hansbuehler/cdxbasics/blob/master/cdxbasics/notebooks/DynamicPlot.ipynb
+ * `close()` to close the figure. If not called, Jupyter creates an unseemly second copy of the graph when the current cell is finished running.
+
+* `color_css4, color_base, color_tableau, color_xkcd`:
+
+ Each function returns the $i$th element of the respective matplotlib color
+ table. The purpose is to simplify using consistent colors accross different plots.
+
+ Example:
+
+ fig = dynaplot.figure()
+ ax = fig.add_subplot()
+ # draw 10 lines in the first sub plot, and add a legend
+ for i in range(10):
+ ax.plot( x, y[i], color=color_css4(i), label=labels[i] )
+ ax.legend()
+ # draw 10 lines in the second sub plot. No legend needed as colors are shared with first plot
+ ax = fig.add_subplot()
+ for i in range(10):
+ ax.plot( x, z[i], color=color_css4(i) )
+ fig.render()
+
+* `colors_css4, colors_base, colors_tableau, colors_xkcd`:
+
+ Generator versions of the `color_` functions.
+
+## prettydict
+
+A number of simple extensions to standard dictionaries which allow accessing any element of the dictionary with "." notation. The purpose is to create a functional-programming style method of generating complex objects.
+
+ from cdxbasics.prettydict import PrettyDict
+ pdct = PrettyDict(z=1)
+ pdct['a'] = 1 # standard dictionary write access
+ pdct.b = 2 # pretty write access
+ _ = pdct.b # read access
+ _ = pdct("c",3) # short cut for pdct.get("c",3)
+
+There are three versions:
+
+* `PrettyDict`:
+ Pretty version of standard dictionary.
+* `PrettyOrderedDict`:
+ Pretty version of ordered dictionary.
+* `PrettySortedDict`:
+ Pretty version of sorted dictionary.
+
+#### Assigning member functions
+
+"Pretty" objects also allow assigning bona fide member functions by a simple semantic of the form:
+
+ def mult_b( self, x ):
+ return self.b * x
+ pdct = mult_a
+
+Calling `pdct.mult_a(3)` with above config will return `6` as expected. This only works when using the member synthax for assigning values
+to a pretty dictionary; if the standard `[]` operator is used then functions will be assigned to the dictionary as usual, hence they are static members of the object.
+
+The reason for this is as follows: consider
+
+ def mult( a, b ):
+ return a*b
+ pdct.mult = mult
+ mult(3,4) --> produces am error as three arguments as are passed if we count 'self'
+
+ In this case, use:
+
+ pdct['mult'] = mult
+ pdct.mult(3,4) --> 12
+
+
+## config
+
+Tooling for setting up program-wide configuration. Aimed at machine learning programs to ensure consistency of code accross experimentation.
+
+ from cdxbasics.config import Config
+ config = Config()
+
+**Key features**
+
+* Detect misspelled parameters by checking that all parameters of a config have been read.
+* Provide summary of all values read, including summary help for what they were for.
+* Nicer synthax than dictionary notation, in particular for nested configurations.
+* Simple validation to ensure values are within a given range or from a list of options.
+
+#### Creating configs
+
+Set data with both dictionary and member notation:
+
+ config = Config()
+ config['features'] = [ 'time', 'spot' ]
+ config.weights = [ 1, 2, 3 ]
+
+Create sub configurations with member notation
+
+ config.network.depth = 10
+ config.network.activation = 'relu'
+ config.network.width = 100
+
+This is equivalent to
+
+ config.network = Config()
+ config.network.depth = 10
+ config.network.activation = 'relu'
+ config.network.width = 100
+
+#### Reading a config
+
+When reading the value of a `key` from config, `config.__call__()` uses a default value, and a cast type. It first attempts to find `key` in the `config`.
+* If `key` is found, it casts the value provided for `key` using the `cast` type and returned.
+* If `key` is not found, then the default value will be cast using `cast` type and returned.
+
+The function also takes a `help` text which allows providing live information on what variable are read from the config. The latter is used by the function `usage_report()` which therefore provides live documentation of the code which uses the config object.
+
+ class Network(object):
+ def __init__( self, config ):
+ # read top level parameters
+ self.features = config("features", [], list, "Features for the agent" )
+ self.weights = config("weights", [], np.asarray, "Weigths for the agent", help_default="no initial weights")
+ config.done() # see below
+
+In above example any data provided for they keywords `weigths` will be cast using `numpy.asarray`.
+
+Further parameters of `()` are the help text, plus ability to provide text versions of the default with `help_default` (e.g. if the default value is complex), and the cast operator with `help_cast` (again if the
+respective operation is complex).
+
+__Important__: the `()` operator does not have a default value unless specified. If no default value is specified, and the key is not found, then a KeyError is generated.
+
+You can read sub-configurations with the previsouly introduced member notation:
+
+ self.activation = config.network("activation", "relu", str, "Activation function for the network")
+
+An alternative is the explicit:
+
+ network = config.network
+ self.depth = network('depth', 10000, int, "Depth for the network")
+
+#### Imposing simple restrictions on values
+
+We can impose simple restrictions to any values read from a config. To this end, import the respective type operators:
+
+ from cdxbasics.config import Int, Float
+
+One-sided restriction:
+
+ # example enforcing simple conditions
+ self.width = network('width', 100, Int>3, "Width for the network")
+
+Restrictions on both sides of a scalar:
+
+ # example encorcing two-sided conditions
+ self.percentage = network('percentage', 0.5, ( Float >= 0. ) & ( Float <= 1.), "A percentage")
+
+Enforce the value being a member of a list:
+
+ # example ensuring a returned type is from a list
+ self.ntype = network('ntype', 'fastforward', ['fastforward','recurrent','lstm'], "Type of network")
+
+We can allow a returned value to be one of several casting types by using tuples. The most common use case is that `None` is a valid value for a config, too. For example, assume that the `name` of the network model should be a string or `None`. This is implemented as
+
+ # example allowing either None or a string
+ self.keras_name = network('name', None, (None, str), "Keras name of the network model")
+
+We can combine conditional expressions with the tuple notation:
+
+ # example allowing either None or a positive int
+ self.batch_size = network('batch_size', None, (None, Int>0), "Batch size or None for TensorFlow's default 32", help_cast="Positive integer, or None")
+
+
+#### Ensuring that we had no typos & that all provided data is meaningful
+
+A common issue when using dictionary-based code is that we might misspell one of the parameters. Unless this is a mandatory parameter we might not notice that we have not actually changed its value in the code below.
+
+To check that all values of `config` are read use `done()`
+
+ config.done() # checks that we have read all keywords.
+
+It will alert you if there are keywords or children which haven't been read. Most likely, those will be typos. Consider the following example where `width` is misspelled in our config:
+
+ class Network(object):
+ def __init__( self, config ):
+ # read top level parameters
+ self.depth = config("depth", 1, Int>=1, "Depth of the network")
+ self.width = config("width", 3, Int>=1, "Width of the network")
+ self.activaton = config("activation", "relu", help="Activation function", help_cast="String with the function name, or function")
+ config.done() # <-- test that all members of config where read
+
+ config = Config()
+ config.features = ['time', 'spot']
+ config.network.depth = 10
+ config.network.activation = 'relu'
+ config.network.widht = 100 # (intentional typo)
+
+ n = Network(config.network)
+
+Since `width` was misspelled in setting up the config, you will get a warning to this end:
+
+ Error closing 'config.network': the following config arguments were not read: ['widht']
+
+ Summary of all variables read from this object:
+ config.network['activation'] = relu # Activation function; default: relu
+ config.network['depth'] = 10 # Depth of the network; default: 1
+ config.network['width'] = 3 # Width of the network; default: 3
+
+Note that you can also call `done()` at top level:
+
+ class Network(object):
+ def __init__( self, config ):
+ # read top level parameters
+ self.depth = config("depth", 1, Int>=1, "Depth of the network")
+ self.width = config("width", 3, Int>=1, "Width of the network")
+ self.activaton = config("activation", "relu", help="Activation function", help_cast="String with the function name, or function")
+
+ config = Config()
+ config.features = ['time', 'spot']
+ config.network.depth = 10
+ config.network.activation = 'relu'
+ config.network.widht = 100 # (intentional typo)
+
+ n = Network(config.network)
+ test_features = config("features", [], list, "Features for my network")
+ config.done()
+
+produces
+
+ ERROR:x:Error closing 'config.network': the following config arguments were not read: ['widht']
+
+ Summary of all variables read from this object:
+ config.network['activation'] = relu # Activation function; default: relu
+ config.network['depth'] = 10 # Depth of the network; default: 1
+ config.network['width'] = 3 # Width of the network; default: 3
+ #
+ config['features'] = ['time', 'spot'] # Default: 2
+
+You can check the status of the use of the config by using the `not_done` property.
+
+
+#### Detaching child configs and other Copy operations
+
+You can also detach a child config, which allows you to store it for later use without triggering `done()` errors:
+
+ def read_config( self, confg ):
+ ...
+ self.config_training = config.training.detach()
+ config.done()
+
+`detach()` will mark he original child as 'done'. Therefore, we will need to call `done()` again, when we finished processing the detached child:
+
+ def training(self)
+ epochs = self.config_training("epochs", 100, int, "Epochs for training")
+ batch_size = self.config_training("batch_size", None, help="Batch size. Use None for default of 32" )
+
+ self.config_training.done()
+
+Use `copy()` to make a bona fide copy of a child, without marking the source child as 'done'. `copy()` will return a config which shares the same status as the source object. If you want an "unused" copy, use `clean_copy()`. A virtual clone is created via `clone()`. A cloned config stores information on usage in the same place for the original object. This is also the semantic of the copy constructor.
+
+#### Self-recording all available configuration parameters
+
+Once your program ran, you can read the summary of all values, their defaults, and their help texts.
+
+ print( config.usage_report( with_cast=True ) )
+
+Prints:
+
+ config.network['activation'] = relu # (str) Activation function for the network; default: relu
+ config.network['depth'] = 10 # (int) Depth for the network; default: 10000
+ config.network['width'] = 100 # (int>3) Width for the network; default: 100
+ config.network['percentage'] = 0.5 # (float>=0. and float<=1.) Width for the network; default: 0.5
+ config.network['ntype'] = 'fastforward' # (['fastforward','recurrent','lstm']) Type of network; default 'fastforward'
+ config.training['batch_size'] = None # () Batch size. Use None for default of 32; default: None
+ config.training['epochs'] = 100 # (int) Epochs for training; default: 100
+ config['features'] = ['time', 'spot'] # (list) Features for the agent; default: []
+ config['weights'] = [1 2 3] # (asarray) Weigths for the agent; default: no initial weights
+
+#### Calling functions with named parameters:
+
+ def create_network( depth=20, activation="relu", width=4 ):
+ ...
+
+We may use
+
+ create_network( **config.network )
+
+However, there is no magic - this function will mark all direct members (not children) as 'done' and will not record the default values of the function `create_network`. Therefore `usage_report` will be somewhat useless. This method will still catch unused variables as "unexpected keyword arguments".
+
+#### Unique ID
+
+Another common use case is that we wish to cache some process in a complex operation. Assuming that the `config` describes all relevant parameters
+we can use `config.unique_id()` to obtain a unique hash ID for the given config.
+
+This can be used, for example, as file name for caching. See also `cdxbasics.subdir` below.
+
+#### Advanced **kwargs Handling
+
+The `Config` class can be used to improve `kwargs` handling.
+Assume we have
+
+ def f(**kwargs):
+ a = kwargs.get("difficult_name", 10)
+ b = kwargs.get("b", 20)
+
+We run the usual risk of somebody mispronouncing the parameter name which we would never know. Therefore we may improve upon the above with
+
+ def f(**kwargs):
+ kwargs = Config(kwargs)
+ a = kwargs("difficult_name", 10)
+ b = kwargs("b", 20)
+ kwargs.done()
+
+If now a user calls `f` with a misspelled `config(difficlt_name=5)` an error will be raised.
+
+Another pattern is to allow both `config` and `kwargs`:
+
+ def f( config=Config(), **kwargs):
+ kwargs = config.detach.update(kwargs)
+ a = kwargs("difficult_name", 10)
+ b = kwargs("b", 20)
+ kwargs.done()
+
+## logger
+
+Tools for defensive programming a'la the C++ ASSERT/VERIFY macros. Aim is to provide one line validation of inputs to functions with intelligible error messages:
+
+ from cdxbasics.logger import Logger
+ _log = Logger(__file__)
+ ...
+ def some_function( a, ...):
+ _log.verify( a==1, "'a' is not one but %s", a)
+ _log.warn_if( a!=1, "'a' was not one but %s", a)
+
+#### Member functions; mostly self-explanatory:
+
+Exceptions independent of logging level
+
+ verify( cond, text, *args, **kwargs )
+ If cond is not met, raise an exception with util.fmt( text, *args, **kwargs ). This is the Python version of C++ VERIFY
+
+ throw_if(cond, text, *args, **kwargs )
+ If cond is met, raise an exception with util.fmt( text, *args, **kwargs )
+
+ throw( text, *args, **kwargs )
+ Just throw an exception with util.fmt( text, *args, **kwargs )
+
+Unconditional logging
+
+ debug( text, *args, **kwargs )
+ info( text, *args, **kwargs )
+ warning( text, *args, **kwargs )
+ error( text, *args, **kwargs )
+ critical( text, *args, **kwargs )
+
+ throw( text, *args, **kwargs )
+
+Verify-conditional functions
+
+ # raise an exception if 'cond' is not True
+ verify( cond, text, *args, **kwargs )
+
+ # print log message of respective level if 'cond' is not True
+ verify_debug( cond, text, *args, **kwargs )
+ verify_info( cond, text, *args, **kwargs )
+ verify_warning( cond, text, *args, **kwargs )
+
+If-conditional functions
+
+ # raise an exception if 'cond' is True
+ throw_if( cond, text, *args, **kwargs )
+
+ # write log message if 'cond' is True
+ debug_if( cond, text, *args, **kwargs )
+ info_if( cond, text, *args, **kwargs )
+ warning_if( cond, text, *args, **kwargs )
+
+ # print message if 'cond' is True
+ prnt_if( cond, text, *args, **kwargs ) # with EOL
+ write_if( cond, text, *args, **kwargs ) # without EOL
+
+## subdir
+
+A few tools to handle file i/o in a transparent way, focusing on caching data. The key idea is to provide transparent, concise pickle access to the file system in a manner similar to dictionary access. Files managed by `subdir` also all have the same extension, which is `pck` by default.
+
+#### Key pattern:
+
+Our pattern assumes that each calcuation is determined by a number of parameters for which we can compute a unique (file) ID for caching results. Unique file IDs can be computed using `uniqueFileName48()`. Here is an example which assumes that `None` is not a valid return value for the underlying function code:
+
+
+ from cdxbasics.config import Config
+ from cdxbasics.subdir import SubDir, CacheMode, uniqueFileName48
+
+ def function_with_caching( config ):
+ # determine caching strategy
+ cache_mode = config.caching("mode", CacheMode.ON, CacheMode.MODES, "Caching strategy: " + CacheMode.HELP)
+ cache_dir = config.caching("directory", "caching", str, "Caching directory")
+ cache_id = config.function.unique_id(length=48)
+
+ # check whether we should delete any existing files
+ if cache_mode.delete:
+ cache_dir.delete(cache_id)
+
+ # read existing file, if desired and possible
+ data_of_my_function = cache_dir.read(cache_id) if cache_mode.read else None
+
+ # check whether we need to compute some data
+ if data_of_my_function is None:
+ ....
+ data_of_my_function = .... use config.function for settings
+ ....
+
+ # write back to disk
+ if cache_node.write:
+ cache_dir.write(cache_id, data_of_my_function)
+
+ return data_of_my_function
+
+See also the example for `CacheMode` below.
+
+
+#### Creating directories
+
+You can create directories using the `SubDir` class. Simply write
+
+ subdir = SubDir("my_directory") # relative to current working directory
+ subdir = SubDir("./my_directory") # relative to current working directory
+ subdir = SubDir("~/my_directory") # relative to home directory
+ subdir = SubDir("!/my_directory") # relative to default temp directory
+
+You can specify a parent for relative path names:
+
+ subdir = SubDir("my_directory", "~") # relative to home directory
+
+Change the extension to `bin`
+
+ subdir = SubDir("~/my_directory;*.bin")
+ subdir = SubDir("~/my_directory", ext="bin")
+ subdir = SubDir("my_directory", "~", ext="bin")
+
+You can also use the `()` operator to generate sub directories. This operator is overloaded: for a single argument, it creates a relative sub-directory:
+
+ parent = SubDir("~/parent")
+ subdir = parent("subdir")
+
+Be aware that when the operator `()` is called with two arguments, then it reads files; see below.
+
+You can obtain a list of all sub directories in a directory by using `subDirs()`.
+
+#### I/O
+##### Reading
+
+To read the data contained in a file 'file.pck' in our subdirectory with extension 'pck' use either of the following
+
+ data = subdir.read("file") # returns the default if file is not found
+ data = subdir.read("file", default=None) # returns the default if file is not found
+
+This function will return `None` by default if 'file' does not exist. You can make it throw an error by calling `subdir.read("file", throwOnError=True)` instead.
+
+You can also use the `()` operator, in which case you must specify a default value (if you don't, then the operator will return a sub directory):
+
+ data = subdir("file", None) # returns None if file is not found
+
+You can also use both member and item notation to access files. In this case, though, an error will be thrown if the file does not exist
+
+ data = subdir.file # raises AtttributeError if file is not found
+ data = subdir['file'] # raises KeyError if file is not found
+
+You can read a range of files in one function call:
+
+ data = subdir.read( ["file1", "file2"] )
+
+Finally, you can also iterate through all existing files:
+
+ for file in subdir:
+ data = subdir.read(file)
+ ...
+
+To obtain a list of all files in our directory which have the correct extension, use `keys()`.
+
+##### Writing
+
+To write data, use any of
+
+ subdir.write("file", data)
+ subdir.file = data
+ subdir['file'] = data
+
+To write several files at once, write
+
+ subdir.write(["file1", "file"], [data1, data2])
+
+Note that when writing to an object, `subdir` will first write to a temporary file, and then rename this file into the target file name. The temporary file name is a `util.uniqueHash48` generated from the target file name, current time, process and thread ID, as well as the machines's UUID. This is done to reduce collisions between processes/machines accessing the same files. It does not remove collision risk entirely, though.
+
+##### Test existence of files
+
+To test existence of 'file' in a directory, use one of
+
+ subdir.exist('file')
+ 'file' in subdir
+
+#### Deleting files
+
+To delete a 'file', use any of the following:
+
+ subdir.delete(file)
+ del subdir.file
+ del subdir['file']
+
+All of these are _silent_, and will not throw errors if 'file' does not exist. In order to throw an error use
+
+ subdir.delete(file, raiseOnError=True)
+
+Other file and directoru deletion methods:
+
+* `deleteAllKeys`: delete all files in the directory, but do not delete sub directories or files with extensions different to our own.
+* `deleteAllContent`: delete all files with our extension, and all sub directories.
+* `eraseEverything`: delete everything
+
+## util
+
+A collection of utility functions.
+
+### uniqueHash
+
+ uniqueHash( *kargs, **kwargs )
+ uniqueHash32( *kargs, **kwargs )
+ uniqueHash48( *kargs, **kwargs )
+ uniqueHash64( *kargs, **kwargs )
+
+Each of these functions returns a unique hash key for the arguments provided for the respective function. The functions *32,*48,*64 return hashes of the respective length, while `uniqueHash` returns the hashes of standard length. These functions will make an effort to robustify the hashes against Python particulars: for example, dictionaries are hashed with sorted keys.
+
+**These functions will ignore all dictionary or object members starting with "`_`".** They also will by default not hash _functions_ or _properties_.
+This is sometimes undesitable, for example when functions are configuration elements:
+
+ config = Config()
+ config.f = lambda x : x**2
+
+
+
+To change this behavuour, use `uniqueHashExt( length : int, parse_functions : bool = False, parse_underscore : str = "nonee")` which returns a hash function of desired lengths with the option to parse elements starting with "`_`" as well.
+
+### CacheMode
+
+A simple enum-type class to help implement a standard caching pattern.
+It implements the following decision matrix
+
+| |on |gen |off |update |clear |readonly|
+|----------------------------------------|------|------|--------|---------|--------|--------|
+|load cache from disk if exists |x |x |- |- |- |x|
+|write updates to disk |x |x |- |x |- |-|
+|delete existing object |- |- |- |- |x |-|
+|delete existing object if incompatible |x |- |- |x |x |-|
+
+Typically, the user is allowed to set the desired `CacheMode` using a `Config` element. The corresponding `CacheMode` object then implements the properties `read`, `write`, `delete` and `del_incomp`.
+
+Prototype code is to be implemented as follows:
+
+ def compute_cached( ..., cache_mode, cache_dir ):
+
+ unique_id = unqiueHash48( ... ) # compute a unique hash for the object
+
+ # delete existing cache if requested
+ if cache_mode.delete:
+ cache_dir.delete(unique_id)
+
+ # attempt to read cache
+ ret = cache_dir.read(unique_id) if cache_mode.read else None
+
+ # validate cache, e.g. is it of the right version
+ if not ret is None:
+ # validate that 'ret is a valid object
+ if not is_valid(ret):
+ if cache_model.del_incomp:
+ cache_dir.delete(unqiue_id)
+ ret = None
+
+ # compute new object if need be
+ if ret is None:
+ # compute new object
+ ret = ...
+
+ # write new object to disk
+ if cache_mode.write:
+ cache_dir.write(unique_id, ret)
+
+ return ret
+
+### WriteLine
+
+A simple utility class to manage printing in a given line with carriage returns (`\r`).
+Essentially, it keeps track of the length what was printed so far at the current line. If a `\r` is encountered it will clear the rest of the line to avoid having residual text from the previous line.
+
+Example 1 (how to use \r and \n)
+
+ write = WriteLine("Initializing...")
+ import time
+ for i in range(10):
+ time.sleep(1)
+ write("\rRunning %g%% ...", round(float(i+1)/float(10)*100,0))
+ write(" done.\nProcess finished.\n")
+
+Example 2 (line length is getting shorter)
+
+ write = WriteLine("Initializing...")
+ import time
+ for i in range(10):
+ time.sleep(1)
+ write("\r" + ("#" * (9-i)))
+ write("\rProcess finished.\n")
+
+### Misc
+
+* `fmt()`: C++ style format function.
+* `plain()`: converts most combinations of standards elements or objects into plain list/dict structures.
+* `isAtomic()`: whether something is string, float, int, bool or date.
+* `isFloat()`: whether something is a float, including a numpy float.
+* `isFunction()`: whether something is some function.
+* `bind()`: simple shortcut to bind function parameters, e.g.
+
+ def f(a, b, c):
+ pass
+ f_a = bind(f, a=1)
+
+* `fmt_list()` returns a nicely formatted list, e.g. `fmt_list([1,2,3])` returns `1, 2 and 3`.
+
+* `fmt_dict()` returns a nicely formatted dictrionary, e.g. `fmt_dict({'a':1,'b':'test'})` returns `a: 1, b: test`.
+* `fmt_seconds()` returns string for seconds, e.g. `fmt_seconds(10)` returns `10s` while `fmt_seconds(61)` returns `1:00`.
+* `fmt_big_number()` converts a large integer into an abbreviated string with terminating `K`, `M`, `B`, `T` as appropriate, using base 10.<br>
+ For example `fmt_big_number(12345)` returns `12.35K`.
+* `fmt_big_byte_number()` converts a large integer into an abbreviated string with terminating `K`, `M`, `G`, `T` as appropriate, here using base 16.<br>For example `fmt_big_byte_number(12345)` returns `12.06K`.
+* `fmt_datetime()` returns a nicely formatted daytime code in natural order e.g. YYYY-MM-DD HH:SS.<br>It returns the respective simplification if just a `date` or `time` is passed instead of a `datetime`.
+
+* `is_jupyter()` tries to assess whether the current environment is a jupyer IPython environment.
+This is experimental as it appears there is no safe way to do this. The current implemenentation checks whether the command which started the current process contains the string `jupyter`.
+
+## np
+
+A small number of statistical numpy functions which take a weight vector (distribution) into account, namely
+
+* `mean(P,x,axis)` computes the mean of `x` using the distribution `P`. If `P` is None, it returns `numpy.mean(x,axis)`.
+* `var(P,x,axis)` computes the variance of `x` using the distribution `P`. If `P` is None, it returns `numpy.var(x,axis)`.
+* `std(P,x,axis)` computes the standard deviation of `x` using the distribution `P`. If `P` is None, it returns `numpy.std(x,axis)`.
+* `err(P,x,axis)` computes the standard error of `x` using the distribution `P`. If `P` is None, it returns `numpy.std(x,axis)/sqrt(x.shape[axis])`.
+
+Two further functions are used to compute binned statistics:
+
+* `mean_bins(x,bins,axis,P)` computes the means of `x` over equidistant `bins` using the distribition `P`.
+* `mean_std_bins(x,bins,axis,P)` computes the means and standard deviations of `x` over equidistant `bins` using the distribition `P`.
+
+
+
+## verbose
+
+**The `verbose` interface has changed in 0.2.36**
+
+This module provides the `Context` utility class for printing 'verbose' information, with indentation depending on the detail level.
+
+The basic idea is that the root context has level 0, with increasing levels for sub-contexts. When printing information, we can (a) limit printing up to a given level and (b) automatically indent the output to reflect the current level of detail.
+
+* Create a `Context` model, and define its verbosity in its constructor, e.g. `all`, `none` or a number. A negative number means that no outout will be generated (`quiet`), while `None` means all output will be printed (`all`). Sub-contexts inherent verbosity from their parents.
+* To write a text at current level to `stdout` use `write()`.
+* To write a text at a sub-level use `report()`. You can also use the overloaded call operator.
+* To create a sub-context, either call `sub()` or use the overloaded call operator.
+
+Here is an example:
+
+ from cdxbasics.verbose import Context, quiet
+
+ def f_sub( num=10, context = quiet ):
+ context.report(0, "Entering loop")
+ for i in range(num):
+ context.report(1, "Number %ld", i)
+
+ def f_main( context = quiet ):
+ context.write( "First step" )
+ # ... do something
+ context.report( 1, "Intermediate step 1" )
+ context.report( 1, "Intermediate step 2\nwith newlines" )
+ # ... do something
+ f_sub( context=context(1) ) # call function f_sub with a sub-context
+ # ... do something
+ context.write( "Final step" )
+
+ print("Verbose=1")
+ context = Context(1)
+ f_main(context)
+
+ print("\nVerbose=2")
+ context = Context(2)
+ f_main(context)
+
+ print("\nVerbose='all'")
+ context = Context('all')
+ f_main(context)
+
+ print("\nVerbose='quiet'")
+ context = Context('quiet')
+ f_main(context)
+
+Returns
+
+ Verbose=1
+ 00: First step
+ 01: Intermediate step 1
+ 01: Intermediate step 2
+ 01: with newlines
+ 01: Entering loop
+ 00: Final step
+
+ Verbose=2
+ 00: First step
+ 01: Intermediate step 1
+ 01: Intermediate step 2
+ 01: with newlines
+ 01: Entering loop
+ 02: Number 0
+ 02: Number 1
+ 02: Number 2
+ 02: Number 3
+ 02: Number 4
+ 02: Number 5
+ 02: Number 6
+ 02: Number 7
+ 02: Number 8
+ 02: Number 9
+ 00: Final step
+
+ Verbose='all'
+ 00: First step
+ 01: Intermediate step 1
+ 01: Intermediate step 2
+ 01: with newlines
+ 01: Entering loop
+ 02: Number 0
+ 02: Number 1
+ 02: Number 2
+ 02: Number 3
+ 02: Number 4
+ 02: Number 5
+ 02: Number 6
+ 02: Number 7
+ 02: Number 8
+ 02: Number 9
+ 00: Final step
+
+ Verbose='quiet'
+
+The purpose of initializing functions usually with `quiet` is that they can be used accross different contexts without printing anything by default.
+
+ + +%package help +Summary: Development documents and examples for cdxbasics +Provides: python3-cdxbasics-doc +%description help +# cdxbasics
+
+Collection of basic tools for Python development.
+
+Install by
+
+ conda install cdxbasics -c hansbuehler
+
+or
+
+ pip install cdxbasics
+
+## dynaplot
+
+Tools for dynamic (animated) plotting in Jupyer/IPython. The aim of the toolkit is making it easy to develop visualization with `matplotlib` which dynamically updates, for example during training with machine learing kits such as `tensorflow`. This has been tested with Anaconda's JupyterHub and `%matplotlib inline`.
+
+Some users reported that the package does not work in some versions of Jupyter. In this case, please try setting `dynaplot.DynamicFig.MODE = 'canvas'`. I appreciate if you let me know whether this resolved
+the problem.
+
+#### Animated Matplotlib in Jupyter
+
+See the jupyter notebook [notebooks/DynamicPlot.ipynb](https://github.com/hansbuehler/cdxbasics/blob/master/cdxbasics/notebooks/DynamicPlot.ipynb) for some applications.
+
+
+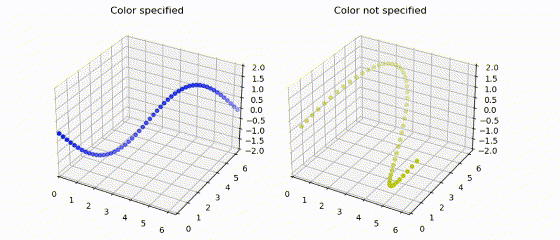
+
+ # example
+ %matplotlib inline
+ import numpy as np
+ x = np.linspace(-5,5,21)
+ y = np.ramdom.normal(size=(21,5))
+
+ # create figure
+ from cdxbasics.dynaplot import figure
+ fig = figure() # equivalent to matplotlib.figure
+ ax = fig.add_subplot() # no need to specify row,col,num
+ l = ax.plot( x, y[:,0] )[0] # get fist line2D object
+ fig.render() # construct figure & draw graph
+
+ # animate
+ import time
+ for i in range(1,5):
+ time.sleep(1)
+ l.set_ydata( y[:,i] ) # update data
+ fig.render()
+
+ fig.close() # clear figure to avoid duplication
+
+See example notebook for how to use the package for lines, confidence intervals, and 3D graphs.
+
+#### Simpler sub_plot
+
+The package lets you create sub plots without having to know the number of plots in advance: you do not need to specify `rol, col, num` when calling `add_subplot`. The underlying figure object will automatically arrange them on a grid for you.
+
+ # create figure
+ from cdxbasics.dynaplot import figure
+ fig = figure(col_size=4, row_size=4, col_num=3)
+ # equivalent to matplotlib.figure
+ ax = fig.add_subplot() # no need to specify row,col,num
+ ax.plot( x, y )
+ ax = fig.add_subplot() # no need to specify row,col,num
+ ax.plot( x, y )
+ ...
+ fig.next_row() # another row
+ ax = fig.add_subplot() # no need to specify row,col,num
+ ax.plot( x, y )
+ ...
+
+ fig.render() # draws the plots
+
+#### Other features
+
+
+
+There are a number of other functions to aid plotting
+
+* `figure()` which returns a `DynamicFig` object:
+
+ Function to replace `matplotlib.figure` which will defer creation of the figure until the first call of `render()`. The effect is that we no longer need to provide the total number of rows and columns in advance - i.e. you won't need to call the equivalent of `fig.add_subplot(3,4,14)` but can just call `fig.add_subplot()`.
+
+ * Instead of `figsize` the function `figure()` accepts `row_size`, `col_size` and `col_nums` to dynamically generate an appropriate figure size.
+
+ Key member functions of `DynamicFig` are:
+ * `add_subplot` to add a new plot. No arguments needed.
+ * `next_row()` to skip to the next row.
+ * `render()` to draw the figure. When called the first time will create all the underlying matplotlib objects. Subsequent calls will re-draw the canvas if the figure was modified. See examples in https://github.com/hansbuehler/cdxbasics/blob/master/cdxbasics/notebooks/DynamicPlot.ipynb
+ * `close()` to close the figure. If not called, Jupyter creates an unseemly second copy of the graph when the current cell is finished running.
+
+* `color_css4, color_base, color_tableau, color_xkcd`:
+
+ Each function returns the $i$th element of the respective matplotlib color
+ table. The purpose is to simplify using consistent colors accross different plots.
+
+ Example:
+
+ fig = dynaplot.figure()
+ ax = fig.add_subplot()
+ # draw 10 lines in the first sub plot, and add a legend
+ for i in range(10):
+ ax.plot( x, y[i], color=color_css4(i), label=labels[i] )
+ ax.legend()
+ # draw 10 lines in the second sub plot. No legend needed as colors are shared with first plot
+ ax = fig.add_subplot()
+ for i in range(10):
+ ax.plot( x, z[i], color=color_css4(i) )
+ fig.render()
+
+* `colors_css4, colors_base, colors_tableau, colors_xkcd`:
+
+ Generator versions of the `color_` functions.
+
+## prettydict
+
+A number of simple extensions to standard dictionaries which allow accessing any element of the dictionary with "." notation. The purpose is to create a functional-programming style method of generating complex objects.
+
+ from cdxbasics.prettydict import PrettyDict
+ pdct = PrettyDict(z=1)
+ pdct['a'] = 1 # standard dictionary write access
+ pdct.b = 2 # pretty write access
+ _ = pdct.b # read access
+ _ = pdct("c",3) # short cut for pdct.get("c",3)
+
+There are three versions:
+
+* `PrettyDict`:
+ Pretty version of standard dictionary.
+* `PrettyOrderedDict`:
+ Pretty version of ordered dictionary.
+* `PrettySortedDict`:
+ Pretty version of sorted dictionary.
+
+#### Assigning member functions
+
+"Pretty" objects also allow assigning bona fide member functions by a simple semantic of the form:
+
+ def mult_b( self, x ):
+ return self.b * x
+ pdct = mult_a
+
+Calling `pdct.mult_a(3)` with above config will return `6` as expected. This only works when using the member synthax for assigning values
+to a pretty dictionary; if the standard `[]` operator is used then functions will be assigned to the dictionary as usual, hence they are static members of the object.
+
+The reason for this is as follows: consider
+
+ def mult( a, b ):
+ return a*b
+ pdct.mult = mult
+ mult(3,4) --> produces am error as three arguments as are passed if we count 'self'
+
+ In this case, use:
+
+ pdct['mult'] = mult
+ pdct.mult(3,4) --> 12
+
+
+## config
+
+Tooling for setting up program-wide configuration. Aimed at machine learning programs to ensure consistency of code accross experimentation.
+
+ from cdxbasics.config import Config
+ config = Config()
+
+**Key features**
+
+* Detect misspelled parameters by checking that all parameters of a config have been read.
+* Provide summary of all values read, including summary help for what they were for.
+* Nicer synthax than dictionary notation, in particular for nested configurations.
+* Simple validation to ensure values are within a given range or from a list of options.
+
+#### Creating configs
+
+Set data with both dictionary and member notation:
+
+ config = Config()
+ config['features'] = [ 'time', 'spot' ]
+ config.weights = [ 1, 2, 3 ]
+
+Create sub configurations with member notation
+
+ config.network.depth = 10
+ config.network.activation = 'relu'
+ config.network.width = 100
+
+This is equivalent to
+
+ config.network = Config()
+ config.network.depth = 10
+ config.network.activation = 'relu'
+ config.network.width = 100
+
+#### Reading a config
+
+When reading the value of a `key` from config, `config.__call__()` uses a default value, and a cast type. It first attempts to find `key` in the `config`.
+* If `key` is found, it casts the value provided for `key` using the `cast` type and returned.
+* If `key` is not found, then the default value will be cast using `cast` type and returned.
+
+The function also takes a `help` text which allows providing live information on what variable are read from the config. The latter is used by the function `usage_report()` which therefore provides live documentation of the code which uses the config object.
+
+ class Network(object):
+ def __init__( self, config ):
+ # read top level parameters
+ self.features = config("features", [], list, "Features for the agent" )
+ self.weights = config("weights", [], np.asarray, "Weigths for the agent", help_default="no initial weights")
+ config.done() # see below
+
+In above example any data provided for they keywords `weigths` will be cast using `numpy.asarray`.
+
+Further parameters of `()` are the help text, plus ability to provide text versions of the default with `help_default` (e.g. if the default value is complex), and the cast operator with `help_cast` (again if the
+respective operation is complex).
+
+__Important__: the `()` operator does not have a default value unless specified. If no default value is specified, and the key is not found, then a KeyError is generated.
+
+You can read sub-configurations with the previsouly introduced member notation:
+
+ self.activation = config.network("activation", "relu", str, "Activation function for the network")
+
+An alternative is the explicit:
+
+ network = config.network
+ self.depth = network('depth', 10000, int, "Depth for the network")
+
+#### Imposing simple restrictions on values
+
+We can impose simple restrictions to any values read from a config. To this end, import the respective type operators:
+
+ from cdxbasics.config import Int, Float
+
+One-sided restriction:
+
+ # example enforcing simple conditions
+ self.width = network('width', 100, Int>3, "Width for the network")
+
+Restrictions on both sides of a scalar:
+
+ # example encorcing two-sided conditions
+ self.percentage = network('percentage', 0.5, ( Float >= 0. ) & ( Float <= 1.), "A percentage")
+
+Enforce the value being a member of a list:
+
+ # example ensuring a returned type is from a list
+ self.ntype = network('ntype', 'fastforward', ['fastforward','recurrent','lstm'], "Type of network")
+
+We can allow a returned value to be one of several casting types by using tuples. The most common use case is that `None` is a valid value for a config, too. For example, assume that the `name` of the network model should be a string or `None`. This is implemented as
+
+ # example allowing either None or a string
+ self.keras_name = network('name', None, (None, str), "Keras name of the network model")
+
+We can combine conditional expressions with the tuple notation:
+
+ # example allowing either None or a positive int
+ self.batch_size = network('batch_size', None, (None, Int>0), "Batch size or None for TensorFlow's default 32", help_cast="Positive integer, or None")
+
+
+#### Ensuring that we had no typos & that all provided data is meaningful
+
+A common issue when using dictionary-based code is that we might misspell one of the parameters. Unless this is a mandatory parameter we might not notice that we have not actually changed its value in the code below.
+
+To check that all values of `config` are read use `done()`
+
+ config.done() # checks that we have read all keywords.
+
+It will alert you if there are keywords or children which haven't been read. Most likely, those will be typos. Consider the following example where `width` is misspelled in our config:
+
+ class Network(object):
+ def __init__( self, config ):
+ # read top level parameters
+ self.depth = config("depth", 1, Int>=1, "Depth of the network")
+ self.width = config("width", 3, Int>=1, "Width of the network")
+ self.activaton = config("activation", "relu", help="Activation function", help_cast="String with the function name, or function")
+ config.done() # <-- test that all members of config where read
+
+ config = Config()
+ config.features = ['time', 'spot']
+ config.network.depth = 10
+ config.network.activation = 'relu'
+ config.network.widht = 100 # (intentional typo)
+
+ n = Network(config.network)
+
+Since `width` was misspelled in setting up the config, you will get a warning to this end:
+
+ Error closing 'config.network': the following config arguments were not read: ['widht']
+
+ Summary of all variables read from this object:
+ config.network['activation'] = relu # Activation function; default: relu
+ config.network['depth'] = 10 # Depth of the network; default: 1
+ config.network['width'] = 3 # Width of the network; default: 3
+
+Note that you can also call `done()` at top level:
+
+ class Network(object):
+ def __init__( self, config ):
+ # read top level parameters
+ self.depth = config("depth", 1, Int>=1, "Depth of the network")
+ self.width = config("width", 3, Int>=1, "Width of the network")
+ self.activaton = config("activation", "relu", help="Activation function", help_cast="String with the function name, or function")
+
+ config = Config()
+ config.features = ['time', 'spot']
+ config.network.depth = 10
+ config.network.activation = 'relu'
+ config.network.widht = 100 # (intentional typo)
+
+ n = Network(config.network)
+ test_features = config("features", [], list, "Features for my network")
+ config.done()
+
+produces
+
+ ERROR:x:Error closing 'config.network': the following config arguments were not read: ['widht']
+
+ Summary of all variables read from this object:
+ config.network['activation'] = relu # Activation function; default: relu
+ config.network['depth'] = 10 # Depth of the network; default: 1
+ config.network['width'] = 3 # Width of the network; default: 3
+ #
+ config['features'] = ['time', 'spot'] # Default: 2
+
+You can check the status of the use of the config by using the `not_done` property.
+
+
+#### Detaching child configs and other Copy operations
+
+You can also detach a child config, which allows you to store it for later use without triggering `done()` errors:
+
+ def read_config( self, confg ):
+ ...
+ self.config_training = config.training.detach()
+ config.done()
+
+`detach()` will mark he original child as 'done'. Therefore, we will need to call `done()` again, when we finished processing the detached child:
+
+ def training(self)
+ epochs = self.config_training("epochs", 100, int, "Epochs for training")
+ batch_size = self.config_training("batch_size", None, help="Batch size. Use None for default of 32" )
+
+ self.config_training.done()
+
+Use `copy()` to make a bona fide copy of a child, without marking the source child as 'done'. `copy()` will return a config which shares the same status as the source object. If you want an "unused" copy, use `clean_copy()`. A virtual clone is created via `clone()`. A cloned config stores information on usage in the same place for the original object. This is also the semantic of the copy constructor.
+
+#### Self-recording all available configuration parameters
+
+Once your program ran, you can read the summary of all values, their defaults, and their help texts.
+
+ print( config.usage_report( with_cast=True ) )
+
+Prints:
+
+ config.network['activation'] = relu # (str) Activation function for the network; default: relu
+ config.network['depth'] = 10 # (int) Depth for the network; default: 10000
+ config.network['width'] = 100 # (int>3) Width for the network; default: 100
+ config.network['percentage'] = 0.5 # (float>=0. and float<=1.) Width for the network; default: 0.5
+ config.network['ntype'] = 'fastforward' # (['fastforward','recurrent','lstm']) Type of network; default 'fastforward'
+ config.training['batch_size'] = None # () Batch size. Use None for default of 32; default: None
+ config.training['epochs'] = 100 # (int) Epochs for training; default: 100
+ config['features'] = ['time', 'spot'] # (list) Features for the agent; default: []
+ config['weights'] = [1 2 3] # (asarray) Weigths for the agent; default: no initial weights
+
+#### Calling functions with named parameters:
+
+ def create_network( depth=20, activation="relu", width=4 ):
+ ...
+
+We may use
+
+ create_network( **config.network )
+
+However, there is no magic - this function will mark all direct members (not children) as 'done' and will not record the default values of the function `create_network`. Therefore `usage_report` will be somewhat useless. This method will still catch unused variables as "unexpected keyword arguments".
+
+#### Unique ID
+
+Another common use case is that we wish to cache some process in a complex operation. Assuming that the `config` describes all relevant parameters
+we can use `config.unique_id()` to obtain a unique hash ID for the given config.
+
+This can be used, for example, as file name for caching. See also `cdxbasics.subdir` below.
+
+#### Advanced **kwargs Handling
+
+The `Config` class can be used to improve `kwargs` handling.
+Assume we have
+
+ def f(**kwargs):
+ a = kwargs.get("difficult_name", 10)
+ b = kwargs.get("b", 20)
+
+We run the usual risk of somebody mispronouncing the parameter name which we would never know. Therefore we may improve upon the above with
+
+ def f(**kwargs):
+ kwargs = Config(kwargs)
+ a = kwargs("difficult_name", 10)
+ b = kwargs("b", 20)
+ kwargs.done()
+
+If now a user calls `f` with a misspelled `config(difficlt_name=5)` an error will be raised.
+
+Another pattern is to allow both `config` and `kwargs`:
+
+ def f( config=Config(), **kwargs):
+ kwargs = config.detach.update(kwargs)
+ a = kwargs("difficult_name", 10)
+ b = kwargs("b", 20)
+ kwargs.done()
+
+## logger
+
+Tools for defensive programming a'la the C++ ASSERT/VERIFY macros. Aim is to provide one line validation of inputs to functions with intelligible error messages:
+
+ from cdxbasics.logger import Logger
+ _log = Logger(__file__)
+ ...
+ def some_function( a, ...):
+ _log.verify( a==1, "'a' is not one but %s", a)
+ _log.warn_if( a!=1, "'a' was not one but %s", a)
+
+#### Member functions; mostly self-explanatory:
+
+Exceptions independent of logging level
+
+ verify( cond, text, *args, **kwargs )
+ If cond is not met, raise an exception with util.fmt( text, *args, **kwargs ). This is the Python version of C++ VERIFY
+
+ throw_if(cond, text, *args, **kwargs )
+ If cond is met, raise an exception with util.fmt( text, *args, **kwargs )
+
+ throw( text, *args, **kwargs )
+ Just throw an exception with util.fmt( text, *args, **kwargs )
+
+Unconditional logging
+
+ debug( text, *args, **kwargs )
+ info( text, *args, **kwargs )
+ warning( text, *args, **kwargs )
+ error( text, *args, **kwargs )
+ critical( text, *args, **kwargs )
+
+ throw( text, *args, **kwargs )
+
+Verify-conditional functions
+
+ # raise an exception if 'cond' is not True
+ verify( cond, text, *args, **kwargs )
+
+ # print log message of respective level if 'cond' is not True
+ verify_debug( cond, text, *args, **kwargs )
+ verify_info( cond, text, *args, **kwargs )
+ verify_warning( cond, text, *args, **kwargs )
+
+If-conditional functions
+
+ # raise an exception if 'cond' is True
+ throw_if( cond, text, *args, **kwargs )
+
+ # write log message if 'cond' is True
+ debug_if( cond, text, *args, **kwargs )
+ info_if( cond, text, *args, **kwargs )
+ warning_if( cond, text, *args, **kwargs )
+
+ # print message if 'cond' is True
+ prnt_if( cond, text, *args, **kwargs ) # with EOL
+ write_if( cond, text, *args, **kwargs ) # without EOL
+
+## subdir
+
+A few tools to handle file i/o in a transparent way, focusing on caching data. The key idea is to provide transparent, concise pickle access to the file system in a manner similar to dictionary access. Files managed by `subdir` also all have the same extension, which is `pck` by default.
+
+#### Key pattern:
+
+Our pattern assumes that each calcuation is determined by a number of parameters for which we can compute a unique (file) ID for caching results. Unique file IDs can be computed using `uniqueFileName48()`. Here is an example which assumes that `None` is not a valid return value for the underlying function code:
+
+
+ from cdxbasics.config import Config
+ from cdxbasics.subdir import SubDir, CacheMode, uniqueFileName48
+
+ def function_with_caching( config ):
+ # determine caching strategy
+ cache_mode = config.caching("mode", CacheMode.ON, CacheMode.MODES, "Caching strategy: " + CacheMode.HELP)
+ cache_dir = config.caching("directory", "caching", str, "Caching directory")
+ cache_id = config.function.unique_id(length=48)
+
+ # check whether we should delete any existing files
+ if cache_mode.delete:
+ cache_dir.delete(cache_id)
+
+ # read existing file, if desired and possible
+ data_of_my_function = cache_dir.read(cache_id) if cache_mode.read else None
+
+ # check whether we need to compute some data
+ if data_of_my_function is None:
+ ....
+ data_of_my_function = .... use config.function for settings
+ ....
+
+ # write back to disk
+ if cache_node.write:
+ cache_dir.write(cache_id, data_of_my_function)
+
+ return data_of_my_function
+
+See also the example for `CacheMode` below.
+
+
+#### Creating directories
+
+You can create directories using the `SubDir` class. Simply write
+
+ subdir = SubDir("my_directory") # relative to current working directory
+ subdir = SubDir("./my_directory") # relative to current working directory
+ subdir = SubDir("~/my_directory") # relative to home directory
+ subdir = SubDir("!/my_directory") # relative to default temp directory
+
+You can specify a parent for relative path names:
+
+ subdir = SubDir("my_directory", "~") # relative to home directory
+
+Change the extension to `bin`
+
+ subdir = SubDir("~/my_directory;*.bin")
+ subdir = SubDir("~/my_directory", ext="bin")
+ subdir = SubDir("my_directory", "~", ext="bin")
+
+You can also use the `()` operator to generate sub directories. This operator is overloaded: for a single argument, it creates a relative sub-directory:
+
+ parent = SubDir("~/parent")
+ subdir = parent("subdir")
+
+Be aware that when the operator `()` is called with two arguments, then it reads files; see below.
+
+You can obtain a list of all sub directories in a directory by using `subDirs()`.
+
+#### I/O
+##### Reading
+
+To read the data contained in a file 'file.pck' in our subdirectory with extension 'pck' use either of the following
+
+ data = subdir.read("file") # returns the default if file is not found
+ data = subdir.read("file", default=None) # returns the default if file is not found
+
+This function will return `None` by default if 'file' does not exist. You can make it throw an error by calling `subdir.read("file", throwOnError=True)` instead.
+
+You can also use the `()` operator, in which case you must specify a default value (if you don't, then the operator will return a sub directory):
+
+ data = subdir("file", None) # returns None if file is not found
+
+You can also use both member and item notation to access files. In this case, though, an error will be thrown if the file does not exist
+
+ data = subdir.file # raises AtttributeError if file is not found
+ data = subdir['file'] # raises KeyError if file is not found
+
+You can read a range of files in one function call:
+
+ data = subdir.read( ["file1", "file2"] )
+
+Finally, you can also iterate through all existing files:
+
+ for file in subdir:
+ data = subdir.read(file)
+ ...
+
+To obtain a list of all files in our directory which have the correct extension, use `keys()`.
+
+##### Writing
+
+To write data, use any of
+
+ subdir.write("file", data)
+ subdir.file = data
+ subdir['file'] = data
+
+To write several files at once, write
+
+ subdir.write(["file1", "file"], [data1, data2])
+
+Note that when writing to an object, `subdir` will first write to a temporary file, and then rename this file into the target file name. The temporary file name is a `util.uniqueHash48` generated from the target file name, current time, process and thread ID, as well as the machines's UUID. This is done to reduce collisions between processes/machines accessing the same files. It does not remove collision risk entirely, though.
+
+##### Test existence of files
+
+To test existence of 'file' in a directory, use one of
+
+ subdir.exist('file')
+ 'file' in subdir
+
+#### Deleting files
+
+To delete a 'file', use any of the following:
+
+ subdir.delete(file)
+ del subdir.file
+ del subdir['file']
+
+All of these are _silent_, and will not throw errors if 'file' does not exist. In order to throw an error use
+
+ subdir.delete(file, raiseOnError=True)
+
+Other file and directoru deletion methods:
+
+* `deleteAllKeys`: delete all files in the directory, but do not delete sub directories or files with extensions different to our own.
+* `deleteAllContent`: delete all files with our extension, and all sub directories.
+* `eraseEverything`: delete everything
+
+## util
+
+A collection of utility functions.
+
+### uniqueHash
+
+ uniqueHash( *kargs, **kwargs )
+ uniqueHash32( *kargs, **kwargs )
+ uniqueHash48( *kargs, **kwargs )
+ uniqueHash64( *kargs, **kwargs )
+
+Each of these functions returns a unique hash key for the arguments provided for the respective function. The functions *32,*48,*64 return hashes of the respective length, while `uniqueHash` returns the hashes of standard length. These functions will make an effort to robustify the hashes against Python particulars: for example, dictionaries are hashed with sorted keys.
+
+**These functions will ignore all dictionary or object members starting with "`_`".** They also will by default not hash _functions_ or _properties_.
+This is sometimes undesitable, for example when functions are configuration elements:
+
+ config = Config()
+ config.f = lambda x : x**2
+
+
+
+To change this behavuour, use `uniqueHashExt( length : int, parse_functions : bool = False, parse_underscore : str = "nonee")` which returns a hash function of desired lengths with the option to parse elements starting with "`_`" as well.
+
+### CacheMode
+
+A simple enum-type class to help implement a standard caching pattern.
+It implements the following decision matrix
+
+| |on |gen |off |update |clear |readonly|
+|----------------------------------------|------|------|--------|---------|--------|--------|
+|load cache from disk if exists |x |x |- |- |- |x|
+|write updates to disk |x |x |- |x |- |-|
+|delete existing object |- |- |- |- |x |-|
+|delete existing object if incompatible |x |- |- |x |x |-|
+
+Typically, the user is allowed to set the desired `CacheMode` using a `Config` element. The corresponding `CacheMode` object then implements the properties `read`, `write`, `delete` and `del_incomp`.
+
+Prototype code is to be implemented as follows:
+
+ def compute_cached( ..., cache_mode, cache_dir ):
+
+ unique_id = unqiueHash48( ... ) # compute a unique hash for the object
+
+ # delete existing cache if requested
+ if cache_mode.delete:
+ cache_dir.delete(unique_id)
+
+ # attempt to read cache
+ ret = cache_dir.read(unique_id) if cache_mode.read else None
+
+ # validate cache, e.g. is it of the right version
+ if not ret is None:
+ # validate that 'ret is a valid object
+ if not is_valid(ret):
+ if cache_model.del_incomp:
+ cache_dir.delete(unqiue_id)
+ ret = None
+
+ # compute new object if need be
+ if ret is None:
+ # compute new object
+ ret = ...
+
+ # write new object to disk
+ if cache_mode.write:
+ cache_dir.write(unique_id, ret)
+
+ return ret
+
+### WriteLine
+
+A simple utility class to manage printing in a given line with carriage returns (`\r`).
+Essentially, it keeps track of the length what was printed so far at the current line. If a `\r` is encountered it will clear the rest of the line to avoid having residual text from the previous line.
+
+Example 1 (how to use \r and \n)
+
+ write = WriteLine("Initializing...")
+ import time
+ for i in range(10):
+ time.sleep(1)
+ write("\rRunning %g%% ...", round(float(i+1)/float(10)*100,0))
+ write(" done.\nProcess finished.\n")
+
+Example 2 (line length is getting shorter)
+
+ write = WriteLine("Initializing...")
+ import time
+ for i in range(10):
+ time.sleep(1)
+ write("\r" + ("#" * (9-i)))
+ write("\rProcess finished.\n")
+
+### Misc
+
+* `fmt()`: C++ style format function.
+* `plain()`: converts most combinations of standards elements or objects into plain list/dict structures.
+* `isAtomic()`: whether something is string, float, int, bool or date.
+* `isFloat()`: whether something is a float, including a numpy float.
+* `isFunction()`: whether something is some function.
+* `bind()`: simple shortcut to bind function parameters, e.g.
+
+ def f(a, b, c):
+ pass
+ f_a = bind(f, a=1)
+
+* `fmt_list()` returns a nicely formatted list, e.g. `fmt_list([1,2,3])` returns `1, 2 and 3`.
+
+* `fmt_dict()` returns a nicely formatted dictrionary, e.g. `fmt_dict({'a':1,'b':'test'})` returns `a: 1, b: test`.
+* `fmt_seconds()` returns string for seconds, e.g. `fmt_seconds(10)` returns `10s` while `fmt_seconds(61)` returns `1:00`.
+* `fmt_big_number()` converts a large integer into an abbreviated string with terminating `K`, `M`, `B`, `T` as appropriate, using base 10.<br>
+ For example `fmt_big_number(12345)` returns `12.35K`.
+* `fmt_big_byte_number()` converts a large integer into an abbreviated string with terminating `K`, `M`, `G`, `T` as appropriate, here using base 16.<br>For example `fmt_big_byte_number(12345)` returns `12.06K`.
+* `fmt_datetime()` returns a nicely formatted daytime code in natural order e.g. YYYY-MM-DD HH:SS.<br>It returns the respective simplification if just a `date` or `time` is passed instead of a `datetime`.
+
+* `is_jupyter()` tries to assess whether the current environment is a jupyer IPython environment.
+This is experimental as it appears there is no safe way to do this. The current implemenentation checks whether the command which started the current process contains the string `jupyter`.
+
+## np
+
+A small number of statistical numpy functions which take a weight vector (distribution) into account, namely
+
+* `mean(P,x,axis)` computes the mean of `x` using the distribution `P`. If `P` is None, it returns `numpy.mean(x,axis)`.
+* `var(P,x,axis)` computes the variance of `x` using the distribution `P`. If `P` is None, it returns `numpy.var(x,axis)`.
+* `std(P,x,axis)` computes the standard deviation of `x` using the distribution `P`. If `P` is None, it returns `numpy.std(x,axis)`.
+* `err(P,x,axis)` computes the standard error of `x` using the distribution `P`. If `P` is None, it returns `numpy.std(x,axis)/sqrt(x.shape[axis])`.
+
+Two further functions are used to compute binned statistics:
+
+* `mean_bins(x,bins,axis,P)` computes the means of `x` over equidistant `bins` using the distribition `P`.
+* `mean_std_bins(x,bins,axis,P)` computes the means and standard deviations of `x` over equidistant `bins` using the distribition `P`.
+
+
+
+## verbose
+
+**The `verbose` interface has changed in 0.2.36**
+
+This module provides the `Context` utility class for printing 'verbose' information, with indentation depending on the detail level.
+
+The basic idea is that the root context has level 0, with increasing levels for sub-contexts. When printing information, we can (a) limit printing up to a given level and (b) automatically indent the output to reflect the current level of detail.
+
+* Create a `Context` model, and define its verbosity in its constructor, e.g. `all`, `none` or a number. A negative number means that no outout will be generated (`quiet`), while `None` means all output will be printed (`all`). Sub-contexts inherent verbosity from their parents.
+* To write a text at current level to `stdout` use `write()`.
+* To write a text at a sub-level use `report()`. You can also use the overloaded call operator.
+* To create a sub-context, either call `sub()` or use the overloaded call operator.
+
+Here is an example:
+
+ from cdxbasics.verbose import Context, quiet
+
+ def f_sub( num=10, context = quiet ):
+ context.report(0, "Entering loop")
+ for i in range(num):
+ context.report(1, "Number %ld", i)
+
+ def f_main( context = quiet ):
+ context.write( "First step" )
+ # ... do something
+ context.report( 1, "Intermediate step 1" )
+ context.report( 1, "Intermediate step 2\nwith newlines" )
+ # ... do something
+ f_sub( context=context(1) ) # call function f_sub with a sub-context
+ # ... do something
+ context.write( "Final step" )
+
+ print("Verbose=1")
+ context = Context(1)
+ f_main(context)
+
+ print("\nVerbose=2")
+ context = Context(2)
+ f_main(context)
+
+ print("\nVerbose='all'")
+ context = Context('all')
+ f_main(context)
+
+ print("\nVerbose='quiet'")
+ context = Context('quiet')
+ f_main(context)
+
+Returns
+
+ Verbose=1
+ 00: First step
+ 01: Intermediate step 1
+ 01: Intermediate step 2
+ 01: with newlines
+ 01: Entering loop
+ 00: Final step
+
+ Verbose=2
+ 00: First step
+ 01: Intermediate step 1
+ 01: Intermediate step 2
+ 01: with newlines
+ 01: Entering loop
+ 02: Number 0
+ 02: Number 1
+ 02: Number 2
+ 02: Number 3
+ 02: Number 4
+ 02: Number 5
+ 02: Number 6
+ 02: Number 7
+ 02: Number 8
+ 02: Number 9
+ 00: Final step
+
+ Verbose='all'
+ 00: First step
+ 01: Intermediate step 1
+ 01: Intermediate step 2
+ 01: with newlines
+ 01: Entering loop
+ 02: Number 0
+ 02: Number 1
+ 02: Number 2
+ 02: Number 3
+ 02: Number 4
+ 02: Number 5
+ 02: Number 6
+ 02: Number 7
+ 02: Number 8
+ 02: Number 9
+ 00: Final step
+
+ Verbose='quiet'
+
+The purpose of initializing functions usually with `quiet` is that they can be used accross different contexts without printing anything by default.
+
+ + +%prep +%autosetup -n cdxbasics-0.2.58 + +%build +%py3_build + +%install +%py3_install +install -d -m755 %{buildroot}/%{_pkgdocdir} +if [ -d doc ]; then cp -arf doc %{buildroot}/%{_pkgdocdir}; fi +if [ -d docs ]; then cp -arf docs %{buildroot}/%{_pkgdocdir}; fi +if [ -d example ]; then cp -arf example %{buildroot}/%{_pkgdocdir}; fi +if [ -d examples ]; then cp -arf examples %{buildroot}/%{_pkgdocdir}; fi +pushd %{buildroot} +if [ -d usr/lib ]; then + find usr/lib -type f -printf "\"/%h/%f\"\n" >> filelist.lst +fi +if [ -d usr/lib64 ]; then + find usr/lib64 -type f -printf "\"/%h/%f\"\n" >> filelist.lst +fi +if [ -d usr/bin ]; then + find usr/bin -type f -printf "\"/%h/%f\"\n" >> filelist.lst +fi +if [ -d usr/sbin ]; then + find usr/sbin -type f -printf "\"/%h/%f\"\n" >> filelist.lst +fi +touch doclist.lst +if [ -d usr/share/man ]; then + find usr/share/man -type f -printf "\"/%h/%f.gz\"\n" >> doclist.lst +fi +popd +mv %{buildroot}/filelist.lst . +mv %{buildroot}/doclist.lst . + +%files -n python3-cdxbasics -f filelist.lst +%dir %{python3_sitelib}/* + +%files help -f doclist.lst +%{_docdir}/* + +%changelog +* Tue Jun 20 2023 Python_Bot <Python_Bot@openeuler.org> - 0.2.58-1 +- Package Spec generated @@ -0,0 +1 @@ +2993cd71fabe745648614a8dd81f4d68 cdxbasics-0.2.58.tar.gz |