1
2
3
4
5
6
7
8
9
10
11
12
13
14
15
16
17
18
19
20
21
22
23
24
25
26
27
28
29
30
31
32
33
34
35
36
37
38
39
40
41
42
43
44
45
46
47
48
49
50
51
52
53
54
55
56
57
58
59
60
61
62
63
64
65
66
67
68
69
70
71
72
73
74
75
76
77
78
79
80
81
82
83
84
85
86
87
88
89
90
91
92
93
94
95
96
97
98
99
100
101
102
103
104
105
106
107
108
109
110
111
112
113
114
115
116
117
118
119
120
121
122
123
124
125
126
127
128
129
130
131
132
133
134
135
136
137
138
139
140
141
142
143
144
145
146
147
148
149
150
151
152
153
154
155
156
157
158
159
160
161
162
163
164
165
166
167
168
169
170
171
172
173
174
175
176
177
178
179
180
181
182
183
184
185
186
187
188
189
190
191
192
193
194
195
196
197
198
199
200
201
202
203
204
205
206
207
208
209
210
211
212
213
214
215
216
217
218
219
220
221
222
223
224
225
226
227
228
229
230
231
232
233
234
235
236
237
238
239
240
241
242
243
244
245
246
247
248
249
250
251
252
253
254
255
256
257
258
259
260
261
262
263
264
265
266
267
268
269
270
271
272
273
274
275
276
277
278
279
280
281
282
283
284
285
286
287
288
289
290
291
292
293
294
295
296
297
298
299
300
301
302
303
304
305
306
307
308
309
310
311
312
313
314
315
316
317
318
319
320
321
322
323
324
325
326
327
328
329
330
331
332
333
334
335
336
337
338
339
340
341
342
343
344
345
346
347
348
349
350
351
352
353
354
355
356
357
358
359
360
361
362
363
364
365
366
367
368
369
370
371
372
373
374
375
376
377
378
379
380
381
382
383
384
385
386
387
388
389
390
391
392
393
394
395
396
397
398
399
400
401
402
403
404
405
406
407
408
409
410
411
412
413
414
415
416
417
418
419
420
421
422
423
424
425
426
427
428
429
430
431
432
433
434
435
436
437
438
439
440
441
442
443
444
445
446
447
448
449
450
451
452
453
454
455
456
457
458
459
460
461
462
463
464
465
466
467
468
469
470
471
472
473
474
475
476
477
478
479
480
481
482
483
484
485
486
487
488
489
490
491
492
493
494
495
496
497
498
499
500
501
502
503
504
505
506
507
508
509
510
511
512
513
514
515
516
517
518
519
520
521
522
523
524
525
526
527
528
529
530
531
532
533
534
535
536
537
538
539
540
|
%global _empty_manifest_terminate_build 0
Name: python-DeepCell
Version: 0.12.5
Release: 1
Summary: Deep learning for single cell image segmentation
License: LICENSE
URL: https://github.com/vanvalenlab/deepcell-tf
Source0: https://mirrors.aliyun.com/pypi/web/packages/f5/40/a0a6c3fe5bce194eeeed7db843a498ebbb0bf762963628cff185d02845c4/DeepCell-0.12.5.tar.gz
BuildArch: noarch
%description
# 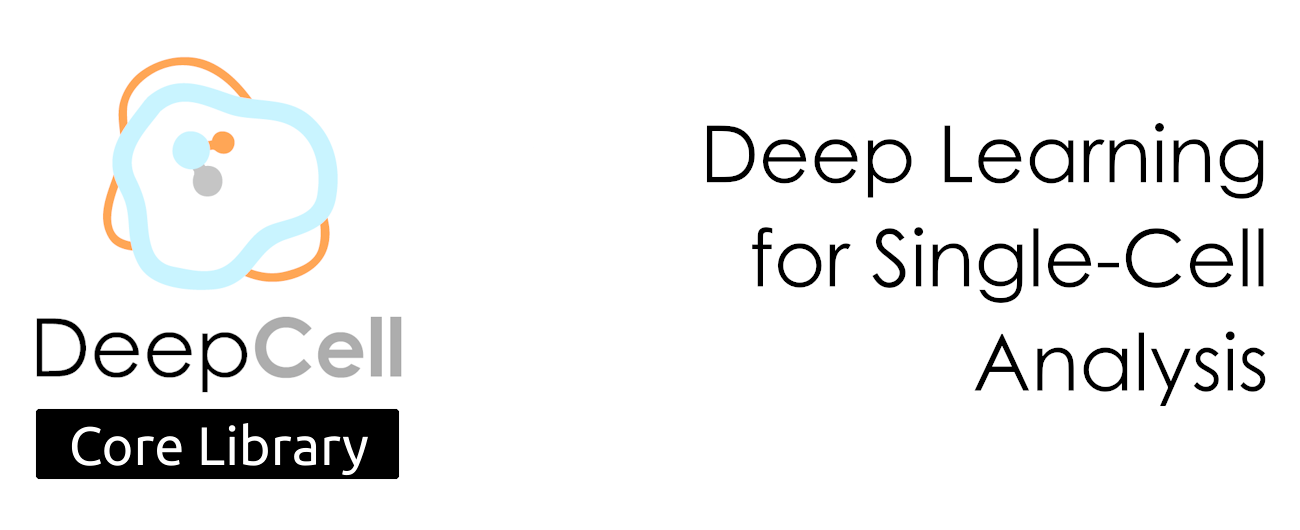
[](https://github.com/vanvalenlab/deepcell-tf/actions)
[](https://coveralls.io/github/vanvalenlab/deepcell-tf?branch=master)
[](https://deepcell.readthedocs.io/en/master/?badge=master)
[](https://github.com/vanvalenlab/deepcell-tf/blob/master/LICENSE)
[](https://badge.fury.io/py/deepcell)
[](https://pypistats.org/packages/deepcell)
[](https://pypi.org/project/deepcell/)
`deepcell-tf` is a deep learning library for single-cell analysis of biological images. It is written in Python and built using [TensorFlow 2](https://github.com/tensorflow/tensorflow).
This library allows users to apply pre-existing models to imaging data as well as to develop new deep learning models for single-cell analysis. This library specializes in models for cell segmentation (whole-cell and nuclear) in 2D and 3D images as well as cell tracking in 2D time-lapse datasets. These models are applicable to data ranging from multiplexed images of tissues to dynamic live-cell imaging movies.
`deepcell-tf` is one of several resources created by the [Van Valen lab](http://vanvalen.caltech.edu/) to facilitate the development and application of new deep learning methods to biology. Other projects within our DeepCell ecosystem include the [DeepCell Toolbox](https://github.com/vanvalenlab/deepcell-toolbox) for pre and post-processing the outputs of deep learning models, [DeepCell Tracking](https://github.com/vanvalenlab/deepcell-tracking) for creating cell lineages with deep-learning-based tracking models, and the [DeepCell Kiosk](https://github.com/vanvalenlab/kiosk-console) for deploying workflows on large datasets in the cloud. Additionally, we have developed [DeepCell Label](https://github.com/vanvalenlab/deepcell-label) for annotating high-dimensional biological images to use as training data.
Read the documentation at [deepcell.readthedocs.io](https://deepcell.readthedocs.io).
For more information on deploying models in the cloud refer to the [the Kiosk documentation](https://deepcell-kiosk.readthedocs.io).
## Examples
<table width="700" border="1" cellpadding="5">
<tr>
<td align="center" valign="center">
Raw Image
</td>
<td align="center" valign="center">
Tracked Image
</td>
</tr>
<tr>
<td align="center" valign="center">
<img src="https://raw.githubusercontent.com/vanvalenlab/deepcell-tf/master/docs/images/raw.gif" alt="Raw Image" />
</td>
<td align="center" valign="center">
<img src="https://raw.githubusercontent.com/vanvalenlab/deepcell-tf/master/docs/images/tracked.gif" alt="Tracked Image" />
</td>
</tr>
</table>
## Getting Started
### Install with pip
The fastest way to get started with `deepcell-tf` is to install the package with `pip`:
```bash
pip install deepcell
```
### Install with Docker
There are also [docker containers](https://hub.docker.com/r/vanvalenlab/deepcell-tf) with GPU support available on DockerHub.
To run the library locally on a GPU, make sure you have [CUDA](https://developer.nvidia.com/cuda-downloads) and [Docker v19.03](https://docs.docker.com/get-docker/) or later installed. For prior Docker versions, use [nvidia-docker](https://github.com/NVIDIA/nvidia-docker).
Alternatively, Google Cloud Platform (GCP) offers prebuilt virtual machines preinstalled with CUDA, Docker, and the NVIDIA Container Toolkit.
Once `docker` is installed, run the following command:
```bash
# Start a GPU enabled container on one GPUs
docker run --gpus '"device=0"' -it --rm \
-p 8888:8888 \
-v $PWD/notebooks:/notebooks \
-v $PWD/data:/data \
vanvalenlab/deepcell-tf:latest-gpu
```
This will start a Docker container with `deepcell-tf` installed and start a jupyter session using the default port 8888. This command also mounts a data folder (`$PWD/data`) and a notebooks folder (`$PWD/notebooks`) to the docker container so it can access data and Juyter notebooks stored on the host workstation. Data and models must be saved in these mounted directories to persist them outside of the running container. The default port can be changed to any non-reserved port by updating `-p 8888:8888` to, e.g., `-p 8080:8888`. If you run across any errors getting started, you should either refer to the `deepcell-tf` for developers section or raise an issue on GitHub.
For examples of how to train models with the `deepcell-tf` library, check out the following notebooks:
- [Training a segmentation model](https://deepcell.readthedocs.io/en/master/notebooks/Training-Segmentation.html)
- [Training a tracking model](https://deepcell.readthedocs.io/en/master/notebooks/Training-Tracking.html)
## DeepCell Applications and DeepCell Datasets
`deepcell-tf` contains two modules that greatly simplify the development and usage of deep learning models for single cell analysis. The first is <tt><a href="https://deepcell.readthedocs.io/en/master/API/deepcell.datasets.html">deepcell.datasets</a></tt>, a collection of biological images that have single-cell annotations. These data include live-cell imaging movies of fluorescent nuclei (approximately 10,000 single-cell trajectories over 30 frames), as well as static images of whole cells (both phase and fluorescence images - approximately 75,000 single cell annotations). The second is <tt><a href="https://deepcell.readthedocs.io/en/master/API/deepcell.applications.html">deepcell.applications</a></tt>, which contains pre-trained models (fluorescent nuclear and phase/fluorescent whole cell) for single-cell analysis. Provided data is scaled so that the physical size of each pixel matches that in the training dataset, these models can be used out of the box on live-cell imaging data. We are currently working to expand these modules to include data and models for tissue images. Please note that they may be spun off into their own GitHub repositories in the near future.
## DeepCell-tf for Developers
`deepcell-tf` uses `docker` and `tensorflow` to enable GPU processing. If using GCP, there are [pre-built images](https://console.cloud.google.com/marketplace/details/nvidia-ngc-public/nvidia_gpu_cloud_image) which come with CUDA and Docker pre-installed. Otherwise, you will need to install [docker](https://docs.docker.com/install/linux/docker-ce/debian/) and [CUDA](https://developer.nvidia.com/cuda-downloads) separately.
### Build a local docker container, specifying the tensorflow version with TF_VERSION
```bash
git clone https://github.com/vanvalenlab/deepcell-tf.git
cd deepcell-tf
docker build --build-arg TF_VERSION=2.8.0-gpu -t $USER/deepcell-tf .
```
### Run the new docker image
```bash
# '"device=0"' refers to the specific GPU(s) to run DeepCell-tf on, and is not required
docker run --gpus '"device=0"' -it \
-p 8888:8888 \
$USER/deepcell-tf:latest-gpu
```
It can also be helpful to mount the local copy of the repository and the notebooks to speed up local development. However, if you are going to mount a local version of the repository, you must first run the docker image without the local repository mounted so that the C extensions can be compiled and then copied over to your local version.
```bash
# First run the docker image without mounting externally
docker run --gpus '"device=0"' -it \
-p 8888:8888 \
$USER/deepcell-tf:latest-gpu
# Use ctrl-p, ctrl-q (or ctrl+p+q) to exit the running docker image without shutting it down
# Then, get the container_id corresponding to the running image of DeepCell-tf
container_id=$(docker ps -q --filter ancestor="$USER/deepcell-tf")
# Copy the compiled c extensions into your local version of the codebase:
docker cp "$container_id:/usr/local/lib/python3.6/dist-packages/deepcell/utils/compute_overlap.cpython-36m-x86_64-linux-gnu.so" deepcell/utils/compute_overlap.cpython-36m-x86_64-linux-gnu.so
# close the running docker
docker kill $container_id
# you can now start the docker image with the code mounted for easy editing
docker run --gpus '"device=0"' -it \
-p 8888:8888 \
-v $PWD/deepcell:/usr/local/lib/python3.6/dist-packages/deepcell/ \
-v $PWD/notebooks:/notebooks \
-v $PWD:/data \
$USER/deepcell-tf:latest-gpu
```
## How to Cite
- [Deep Learning Automates the Quantitative Analysis of Individual Cells in Live-Cell Imaging Experiments](https://journals.plos.org/ploscompbiol/article?id=10.1371/journal.pcbi.1005177)
- [Accurate cell tracking and lineage construction in live-cell imaging experiments with deep learning](https://www.biorxiv.org/content/10.1101/803205v2)
- [DeepCell Kiosk: scaling deep learning–enabled cellular image analysis with Kubernetes](https://www.nature.com/articles/s41592-020-01023-0)
- [Whole-cell segmentation of tissue images with human-level performance using large-scale data annotation and deep learning](https://www.nature.com/articles/s41587-021-01094-0)
## Copyright
Copyright © 2016-2023 [The Van Valen Lab](http://www.vanvalen.caltech.edu/) at the California Institute of Technology (Caltech), with support from the Shurl and Kay Curci Foundation, Google Research Cloud, the Paul Allen Family Foundation, & National Institutes of Health (NIH) under Grant U24CA224309-01.
All rights reserved.
## License
This software is licensed under a modified [APACHE2](https://github.com/vanvalenlab/deepcell-tf/blob/master/LICENSE). See [LICENSE](https://github.com/vanvalenlab/deepcell-tf/blob/master/LICENSE) for full details.
## Trademarks
All other trademarks referenced herein are the property of their respective owners.
## Credits
[](http://www.vanvalen.caltech.edu/)
%package -n python3-DeepCell
Summary: Deep learning for single cell image segmentation
Provides: python-DeepCell
BuildRequires: python3-devel
BuildRequires: python3-setuptools
BuildRequires: python3-pip
%description -n python3-DeepCell
# 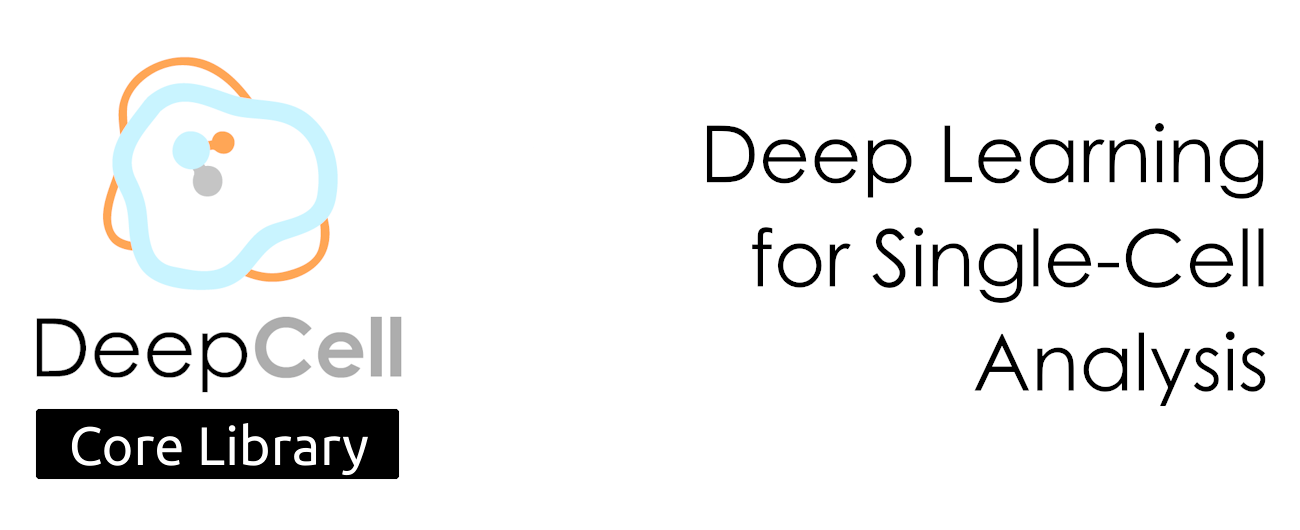
[](https://github.com/vanvalenlab/deepcell-tf/actions)
[](https://coveralls.io/github/vanvalenlab/deepcell-tf?branch=master)
[](https://deepcell.readthedocs.io/en/master/?badge=master)
[](https://github.com/vanvalenlab/deepcell-tf/blob/master/LICENSE)
[](https://badge.fury.io/py/deepcell)
[](https://pypistats.org/packages/deepcell)
[](https://pypi.org/project/deepcell/)
`deepcell-tf` is a deep learning library for single-cell analysis of biological images. It is written in Python and built using [TensorFlow 2](https://github.com/tensorflow/tensorflow).
This library allows users to apply pre-existing models to imaging data as well as to develop new deep learning models for single-cell analysis. This library specializes in models for cell segmentation (whole-cell and nuclear) in 2D and 3D images as well as cell tracking in 2D time-lapse datasets. These models are applicable to data ranging from multiplexed images of tissues to dynamic live-cell imaging movies.
`deepcell-tf` is one of several resources created by the [Van Valen lab](http://vanvalen.caltech.edu/) to facilitate the development and application of new deep learning methods to biology. Other projects within our DeepCell ecosystem include the [DeepCell Toolbox](https://github.com/vanvalenlab/deepcell-toolbox) for pre and post-processing the outputs of deep learning models, [DeepCell Tracking](https://github.com/vanvalenlab/deepcell-tracking) for creating cell lineages with deep-learning-based tracking models, and the [DeepCell Kiosk](https://github.com/vanvalenlab/kiosk-console) for deploying workflows on large datasets in the cloud. Additionally, we have developed [DeepCell Label](https://github.com/vanvalenlab/deepcell-label) for annotating high-dimensional biological images to use as training data.
Read the documentation at [deepcell.readthedocs.io](https://deepcell.readthedocs.io).
For more information on deploying models in the cloud refer to the [the Kiosk documentation](https://deepcell-kiosk.readthedocs.io).
## Examples
<table width="700" border="1" cellpadding="5">
<tr>
<td align="center" valign="center">
Raw Image
</td>
<td align="center" valign="center">
Tracked Image
</td>
</tr>
<tr>
<td align="center" valign="center">
<img src="https://raw.githubusercontent.com/vanvalenlab/deepcell-tf/master/docs/images/raw.gif" alt="Raw Image" />
</td>
<td align="center" valign="center">
<img src="https://raw.githubusercontent.com/vanvalenlab/deepcell-tf/master/docs/images/tracked.gif" alt="Tracked Image" />
</td>
</tr>
</table>
## Getting Started
### Install with pip
The fastest way to get started with `deepcell-tf` is to install the package with `pip`:
```bash
pip install deepcell
```
### Install with Docker
There are also [docker containers](https://hub.docker.com/r/vanvalenlab/deepcell-tf) with GPU support available on DockerHub.
To run the library locally on a GPU, make sure you have [CUDA](https://developer.nvidia.com/cuda-downloads) and [Docker v19.03](https://docs.docker.com/get-docker/) or later installed. For prior Docker versions, use [nvidia-docker](https://github.com/NVIDIA/nvidia-docker).
Alternatively, Google Cloud Platform (GCP) offers prebuilt virtual machines preinstalled with CUDA, Docker, and the NVIDIA Container Toolkit.
Once `docker` is installed, run the following command:
```bash
# Start a GPU enabled container on one GPUs
docker run --gpus '"device=0"' -it --rm \
-p 8888:8888 \
-v $PWD/notebooks:/notebooks \
-v $PWD/data:/data \
vanvalenlab/deepcell-tf:latest-gpu
```
This will start a Docker container with `deepcell-tf` installed and start a jupyter session using the default port 8888. This command also mounts a data folder (`$PWD/data`) and a notebooks folder (`$PWD/notebooks`) to the docker container so it can access data and Juyter notebooks stored on the host workstation. Data and models must be saved in these mounted directories to persist them outside of the running container. The default port can be changed to any non-reserved port by updating `-p 8888:8888` to, e.g., `-p 8080:8888`. If you run across any errors getting started, you should either refer to the `deepcell-tf` for developers section or raise an issue on GitHub.
For examples of how to train models with the `deepcell-tf` library, check out the following notebooks:
- [Training a segmentation model](https://deepcell.readthedocs.io/en/master/notebooks/Training-Segmentation.html)
- [Training a tracking model](https://deepcell.readthedocs.io/en/master/notebooks/Training-Tracking.html)
## DeepCell Applications and DeepCell Datasets
`deepcell-tf` contains two modules that greatly simplify the development and usage of deep learning models for single cell analysis. The first is <tt><a href="https://deepcell.readthedocs.io/en/master/API/deepcell.datasets.html">deepcell.datasets</a></tt>, a collection of biological images that have single-cell annotations. These data include live-cell imaging movies of fluorescent nuclei (approximately 10,000 single-cell trajectories over 30 frames), as well as static images of whole cells (both phase and fluorescence images - approximately 75,000 single cell annotations). The second is <tt><a href="https://deepcell.readthedocs.io/en/master/API/deepcell.applications.html">deepcell.applications</a></tt>, which contains pre-trained models (fluorescent nuclear and phase/fluorescent whole cell) for single-cell analysis. Provided data is scaled so that the physical size of each pixel matches that in the training dataset, these models can be used out of the box on live-cell imaging data. We are currently working to expand these modules to include data and models for tissue images. Please note that they may be spun off into their own GitHub repositories in the near future.
## DeepCell-tf for Developers
`deepcell-tf` uses `docker` and `tensorflow` to enable GPU processing. If using GCP, there are [pre-built images](https://console.cloud.google.com/marketplace/details/nvidia-ngc-public/nvidia_gpu_cloud_image) which come with CUDA and Docker pre-installed. Otherwise, you will need to install [docker](https://docs.docker.com/install/linux/docker-ce/debian/) and [CUDA](https://developer.nvidia.com/cuda-downloads) separately.
### Build a local docker container, specifying the tensorflow version with TF_VERSION
```bash
git clone https://github.com/vanvalenlab/deepcell-tf.git
cd deepcell-tf
docker build --build-arg TF_VERSION=2.8.0-gpu -t $USER/deepcell-tf .
```
### Run the new docker image
```bash
# '"device=0"' refers to the specific GPU(s) to run DeepCell-tf on, and is not required
docker run --gpus '"device=0"' -it \
-p 8888:8888 \
$USER/deepcell-tf:latest-gpu
```
It can also be helpful to mount the local copy of the repository and the notebooks to speed up local development. However, if you are going to mount a local version of the repository, you must first run the docker image without the local repository mounted so that the C extensions can be compiled and then copied over to your local version.
```bash
# First run the docker image without mounting externally
docker run --gpus '"device=0"' -it \
-p 8888:8888 \
$USER/deepcell-tf:latest-gpu
# Use ctrl-p, ctrl-q (or ctrl+p+q) to exit the running docker image without shutting it down
# Then, get the container_id corresponding to the running image of DeepCell-tf
container_id=$(docker ps -q --filter ancestor="$USER/deepcell-tf")
# Copy the compiled c extensions into your local version of the codebase:
docker cp "$container_id:/usr/local/lib/python3.6/dist-packages/deepcell/utils/compute_overlap.cpython-36m-x86_64-linux-gnu.so" deepcell/utils/compute_overlap.cpython-36m-x86_64-linux-gnu.so
# close the running docker
docker kill $container_id
# you can now start the docker image with the code mounted for easy editing
docker run --gpus '"device=0"' -it \
-p 8888:8888 \
-v $PWD/deepcell:/usr/local/lib/python3.6/dist-packages/deepcell/ \
-v $PWD/notebooks:/notebooks \
-v $PWD:/data \
$USER/deepcell-tf:latest-gpu
```
## How to Cite
- [Deep Learning Automates the Quantitative Analysis of Individual Cells in Live-Cell Imaging Experiments](https://journals.plos.org/ploscompbiol/article?id=10.1371/journal.pcbi.1005177)
- [Accurate cell tracking and lineage construction in live-cell imaging experiments with deep learning](https://www.biorxiv.org/content/10.1101/803205v2)
- [DeepCell Kiosk: scaling deep learning–enabled cellular image analysis with Kubernetes](https://www.nature.com/articles/s41592-020-01023-0)
- [Whole-cell segmentation of tissue images with human-level performance using large-scale data annotation and deep learning](https://www.nature.com/articles/s41587-021-01094-0)
## Copyright
Copyright © 2016-2023 [The Van Valen Lab](http://www.vanvalen.caltech.edu/) at the California Institute of Technology (Caltech), with support from the Shurl and Kay Curci Foundation, Google Research Cloud, the Paul Allen Family Foundation, & National Institutes of Health (NIH) under Grant U24CA224309-01.
All rights reserved.
## License
This software is licensed under a modified [APACHE2](https://github.com/vanvalenlab/deepcell-tf/blob/master/LICENSE). See [LICENSE](https://github.com/vanvalenlab/deepcell-tf/blob/master/LICENSE) for full details.
## Trademarks
All other trademarks referenced herein are the property of their respective owners.
## Credits
[](http://www.vanvalen.caltech.edu/)
%package help
Summary: Development documents and examples for DeepCell
Provides: python3-DeepCell-doc
%description help
# 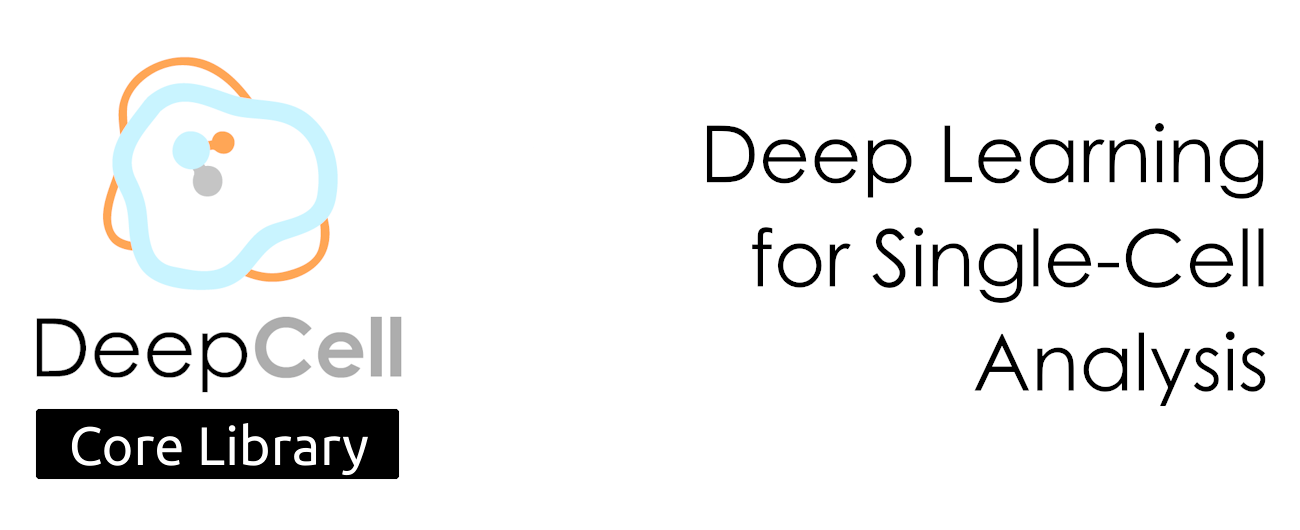
[](https://github.com/vanvalenlab/deepcell-tf/actions)
[](https://coveralls.io/github/vanvalenlab/deepcell-tf?branch=master)
[](https://deepcell.readthedocs.io/en/master/?badge=master)
[](https://github.com/vanvalenlab/deepcell-tf/blob/master/LICENSE)
[](https://badge.fury.io/py/deepcell)
[](https://pypistats.org/packages/deepcell)
[](https://pypi.org/project/deepcell/)
`deepcell-tf` is a deep learning library for single-cell analysis of biological images. It is written in Python and built using [TensorFlow 2](https://github.com/tensorflow/tensorflow).
This library allows users to apply pre-existing models to imaging data as well as to develop new deep learning models for single-cell analysis. This library specializes in models for cell segmentation (whole-cell and nuclear) in 2D and 3D images as well as cell tracking in 2D time-lapse datasets. These models are applicable to data ranging from multiplexed images of tissues to dynamic live-cell imaging movies.
`deepcell-tf` is one of several resources created by the [Van Valen lab](http://vanvalen.caltech.edu/) to facilitate the development and application of new deep learning methods to biology. Other projects within our DeepCell ecosystem include the [DeepCell Toolbox](https://github.com/vanvalenlab/deepcell-toolbox) for pre and post-processing the outputs of deep learning models, [DeepCell Tracking](https://github.com/vanvalenlab/deepcell-tracking) for creating cell lineages with deep-learning-based tracking models, and the [DeepCell Kiosk](https://github.com/vanvalenlab/kiosk-console) for deploying workflows on large datasets in the cloud. Additionally, we have developed [DeepCell Label](https://github.com/vanvalenlab/deepcell-label) for annotating high-dimensional biological images to use as training data.
Read the documentation at [deepcell.readthedocs.io](https://deepcell.readthedocs.io).
For more information on deploying models in the cloud refer to the [the Kiosk documentation](https://deepcell-kiosk.readthedocs.io).
## Examples
<table width="700" border="1" cellpadding="5">
<tr>
<td align="center" valign="center">
Raw Image
</td>
<td align="center" valign="center">
Tracked Image
</td>
</tr>
<tr>
<td align="center" valign="center">
<img src="https://raw.githubusercontent.com/vanvalenlab/deepcell-tf/master/docs/images/raw.gif" alt="Raw Image" />
</td>
<td align="center" valign="center">
<img src="https://raw.githubusercontent.com/vanvalenlab/deepcell-tf/master/docs/images/tracked.gif" alt="Tracked Image" />
</td>
</tr>
</table>
## Getting Started
### Install with pip
The fastest way to get started with `deepcell-tf` is to install the package with `pip`:
```bash
pip install deepcell
```
### Install with Docker
There are also [docker containers](https://hub.docker.com/r/vanvalenlab/deepcell-tf) with GPU support available on DockerHub.
To run the library locally on a GPU, make sure you have [CUDA](https://developer.nvidia.com/cuda-downloads) and [Docker v19.03](https://docs.docker.com/get-docker/) or later installed. For prior Docker versions, use [nvidia-docker](https://github.com/NVIDIA/nvidia-docker).
Alternatively, Google Cloud Platform (GCP) offers prebuilt virtual machines preinstalled with CUDA, Docker, and the NVIDIA Container Toolkit.
Once `docker` is installed, run the following command:
```bash
# Start a GPU enabled container on one GPUs
docker run --gpus '"device=0"' -it --rm \
-p 8888:8888 \
-v $PWD/notebooks:/notebooks \
-v $PWD/data:/data \
vanvalenlab/deepcell-tf:latest-gpu
```
This will start a Docker container with `deepcell-tf` installed and start a jupyter session using the default port 8888. This command also mounts a data folder (`$PWD/data`) and a notebooks folder (`$PWD/notebooks`) to the docker container so it can access data and Juyter notebooks stored on the host workstation. Data and models must be saved in these mounted directories to persist them outside of the running container. The default port can be changed to any non-reserved port by updating `-p 8888:8888` to, e.g., `-p 8080:8888`. If you run across any errors getting started, you should either refer to the `deepcell-tf` for developers section or raise an issue on GitHub.
For examples of how to train models with the `deepcell-tf` library, check out the following notebooks:
- [Training a segmentation model](https://deepcell.readthedocs.io/en/master/notebooks/Training-Segmentation.html)
- [Training a tracking model](https://deepcell.readthedocs.io/en/master/notebooks/Training-Tracking.html)
## DeepCell Applications and DeepCell Datasets
`deepcell-tf` contains two modules that greatly simplify the development and usage of deep learning models for single cell analysis. The first is <tt><a href="https://deepcell.readthedocs.io/en/master/API/deepcell.datasets.html">deepcell.datasets</a></tt>, a collection of biological images that have single-cell annotations. These data include live-cell imaging movies of fluorescent nuclei (approximately 10,000 single-cell trajectories over 30 frames), as well as static images of whole cells (both phase and fluorescence images - approximately 75,000 single cell annotations). The second is <tt><a href="https://deepcell.readthedocs.io/en/master/API/deepcell.applications.html">deepcell.applications</a></tt>, which contains pre-trained models (fluorescent nuclear and phase/fluorescent whole cell) for single-cell analysis. Provided data is scaled so that the physical size of each pixel matches that in the training dataset, these models can be used out of the box on live-cell imaging data. We are currently working to expand these modules to include data and models for tissue images. Please note that they may be spun off into their own GitHub repositories in the near future.
## DeepCell-tf for Developers
`deepcell-tf` uses `docker` and `tensorflow` to enable GPU processing. If using GCP, there are [pre-built images](https://console.cloud.google.com/marketplace/details/nvidia-ngc-public/nvidia_gpu_cloud_image) which come with CUDA and Docker pre-installed. Otherwise, you will need to install [docker](https://docs.docker.com/install/linux/docker-ce/debian/) and [CUDA](https://developer.nvidia.com/cuda-downloads) separately.
### Build a local docker container, specifying the tensorflow version with TF_VERSION
```bash
git clone https://github.com/vanvalenlab/deepcell-tf.git
cd deepcell-tf
docker build --build-arg TF_VERSION=2.8.0-gpu -t $USER/deepcell-tf .
```
### Run the new docker image
```bash
# '"device=0"' refers to the specific GPU(s) to run DeepCell-tf on, and is not required
docker run --gpus '"device=0"' -it \
-p 8888:8888 \
$USER/deepcell-tf:latest-gpu
```
It can also be helpful to mount the local copy of the repository and the notebooks to speed up local development. However, if you are going to mount a local version of the repository, you must first run the docker image without the local repository mounted so that the C extensions can be compiled and then copied over to your local version.
```bash
# First run the docker image without mounting externally
docker run --gpus '"device=0"' -it \
-p 8888:8888 \
$USER/deepcell-tf:latest-gpu
# Use ctrl-p, ctrl-q (or ctrl+p+q) to exit the running docker image without shutting it down
# Then, get the container_id corresponding to the running image of DeepCell-tf
container_id=$(docker ps -q --filter ancestor="$USER/deepcell-tf")
# Copy the compiled c extensions into your local version of the codebase:
docker cp "$container_id:/usr/local/lib/python3.6/dist-packages/deepcell/utils/compute_overlap.cpython-36m-x86_64-linux-gnu.so" deepcell/utils/compute_overlap.cpython-36m-x86_64-linux-gnu.so
# close the running docker
docker kill $container_id
# you can now start the docker image with the code mounted for easy editing
docker run --gpus '"device=0"' -it \
-p 8888:8888 \
-v $PWD/deepcell:/usr/local/lib/python3.6/dist-packages/deepcell/ \
-v $PWD/notebooks:/notebooks \
-v $PWD:/data \
$USER/deepcell-tf:latest-gpu
```
## How to Cite
- [Deep Learning Automates the Quantitative Analysis of Individual Cells in Live-Cell Imaging Experiments](https://journals.plos.org/ploscompbiol/article?id=10.1371/journal.pcbi.1005177)
- [Accurate cell tracking and lineage construction in live-cell imaging experiments with deep learning](https://www.biorxiv.org/content/10.1101/803205v2)
- [DeepCell Kiosk: scaling deep learning–enabled cellular image analysis with Kubernetes](https://www.nature.com/articles/s41592-020-01023-0)
- [Whole-cell segmentation of tissue images with human-level performance using large-scale data annotation and deep learning](https://www.nature.com/articles/s41587-021-01094-0)
## Copyright
Copyright © 2016-2023 [The Van Valen Lab](http://www.vanvalen.caltech.edu/) at the California Institute of Technology (Caltech), with support from the Shurl and Kay Curci Foundation, Google Research Cloud, the Paul Allen Family Foundation, & National Institutes of Health (NIH) under Grant U24CA224309-01.
All rights reserved.
## License
This software is licensed under a modified [APACHE2](https://github.com/vanvalenlab/deepcell-tf/blob/master/LICENSE). See [LICENSE](https://github.com/vanvalenlab/deepcell-tf/blob/master/LICENSE) for full details.
## Trademarks
All other trademarks referenced herein are the property of their respective owners.
## Credits
[](http://www.vanvalen.caltech.edu/)
%prep
%autosetup -n DeepCell-0.12.5
%build
%py3_build
%install
%py3_install
install -d -m755 %{buildroot}/%{_pkgdocdir}
if [ -d doc ]; then cp -arf doc %{buildroot}/%{_pkgdocdir}; fi
if [ -d docs ]; then cp -arf docs %{buildroot}/%{_pkgdocdir}; fi
if [ -d example ]; then cp -arf example %{buildroot}/%{_pkgdocdir}; fi
if [ -d examples ]; then cp -arf examples %{buildroot}/%{_pkgdocdir}; fi
pushd %{buildroot}
if [ -d usr/lib ]; then
find usr/lib -type f -printf "\"/%h/%f\"\n" >> filelist.lst
fi
if [ -d usr/lib64 ]; then
find usr/lib64 -type f -printf "\"/%h/%f\"\n" >> filelist.lst
fi
if [ -d usr/bin ]; then
find usr/bin -type f -printf "\"/%h/%f\"\n" >> filelist.lst
fi
if [ -d usr/sbin ]; then
find usr/sbin -type f -printf "\"/%h/%f\"\n" >> filelist.lst
fi
touch doclist.lst
if [ -d usr/share/man ]; then
find usr/share/man -type f -printf "\"/%h/%f.gz\"\n" >> doclist.lst
fi
popd
mv %{buildroot}/filelist.lst .
mv %{buildroot}/doclist.lst .
%files -n python3-DeepCell -f filelist.lst
%dir %{python3_sitelib}/*
%files help -f doclist.lst
%{_docdir}/*
%changelog
* Thu Jun 08 2023 Python_Bot <Python_Bot@openeuler.org> - 0.12.5-1
- Package Spec generated
|