1
2
3
4
5
6
7
8
9
10
11
12
13
14
15
16
17
18
19
20
21
22
23
24
25
26
27
28
29
30
31
32
33
34
35
36
37
38
39
40
41
42
43
44
45
46
47
48
49
50
51
52
53
54
55
56
57
58
59
60
61
62
63
64
65
66
67
68
69
70
71
72
73
74
75
76
77
78
79
80
81
82
83
84
85
86
87
88
89
90
91
92
93
94
95
96
97
98
99
100
101
102
103
104
105
106
107
108
109
110
111
112
113
114
115
116
117
118
119
120
121
122
123
124
125
126
127
128
129
130
131
132
133
134
135
136
137
138
139
140
141
142
143
144
145
146
147
148
149
150
151
152
153
154
155
156
157
158
159
160
161
162
163
164
165
166
167
168
169
170
171
172
173
174
175
176
177
178
179
180
181
182
183
184
185
186
187
188
189
190
191
192
193
194
195
196
197
198
199
200
201
202
203
204
205
206
207
208
209
210
211
212
213
214
215
216
217
218
219
220
221
222
223
224
225
226
227
228
229
230
231
232
233
234
235
236
237
238
239
240
241
242
243
244
245
246
247
248
249
250
251
252
253
254
255
256
257
258
259
260
261
262
263
264
265
266
267
268
269
270
271
272
273
274
275
276
277
278
279
280
281
282
283
284
285
286
287
288
289
290
291
292
293
294
295
296
297
298
299
300
301
302
303
304
305
306
307
308
309
310
311
312
313
314
315
316
317
318
319
320
321
322
323
324
325
326
327
328
329
330
331
332
333
334
335
336
337
338
339
340
341
342
343
344
345
346
347
348
349
350
351
352
353
354
355
356
357
358
359
360
361
362
363
364
365
366
367
368
369
370
371
372
373
374
375
376
377
378
379
380
381
382
383
384
385
386
387
388
389
390
391
392
393
394
395
396
397
398
399
400
401
402
403
404
405
406
407
408
409
410
411
412
413
414
415
416
417
418
419
420
421
422
423
424
425
426
427
428
429
430
431
432
433
434
435
436
437
438
439
440
441
442
443
444
445
446
447
448
449
450
451
452
453
454
455
456
457
458
459
460
461
462
463
464
465
466
467
468
469
470
471
472
473
474
475
476
477
478
479
480
481
482
483
484
485
486
487
488
489
490
491
492
493
494
495
496
497
498
499
500
501
502
503
504
505
506
507
508
509
510
511
512
513
514
515
516
517
518
519
520
521
522
523
524
525
526
527
528
529
530
531
532
533
534
535
536
537
538
539
540
541
542
543
544
545
546
547
548
549
550
551
552
553
554
555
556
557
558
559
560
561
562
563
564
565
566
567
568
569
570
571
572
573
574
575
576
577
578
579
580
581
582
583
584
585
586
587
588
589
590
591
592
593
594
595
596
597
598
599
600
601
602
603
604
605
606
607
608
609
610
611
612
613
614
615
616
617
618
619
620
621
622
623
624
625
626
627
628
629
630
631
632
633
634
635
636
637
638
639
640
641
642
643
644
645
646
647
648
649
650
651
652
653
654
655
656
657
658
659
660
661
662
663
664
665
666
667
668
669
670
671
672
673
674
675
676
677
678
679
680
|
%global _empty_manifest_terminate_build 0
Name: python-norfair
Version: 2.2.0
Release: 1
Summary: Lightweight Python library for adding real-time multi-object tracking to any detector.
License: BSD-3-Clause
URL: https://github.com/tryolabs/norfair
Source0: https://mirrors.nju.edu.cn/pypi/web/packages/cf/8a/5da8515bdbbe44d40bff5341e12ae1269963d48275f468677ac2ce649c51/norfair-2.2.0.tar.gz
BuildArch: noarch
Requires: python3-filterpy
Requires: python3-rich
Requires: python3-scipy
Requires: python3-numpy
Requires: python3-numpy
Requires: python3-opencv-python
Requires: python3-motmetrics
Requires: python3-importlib-metadata
%description
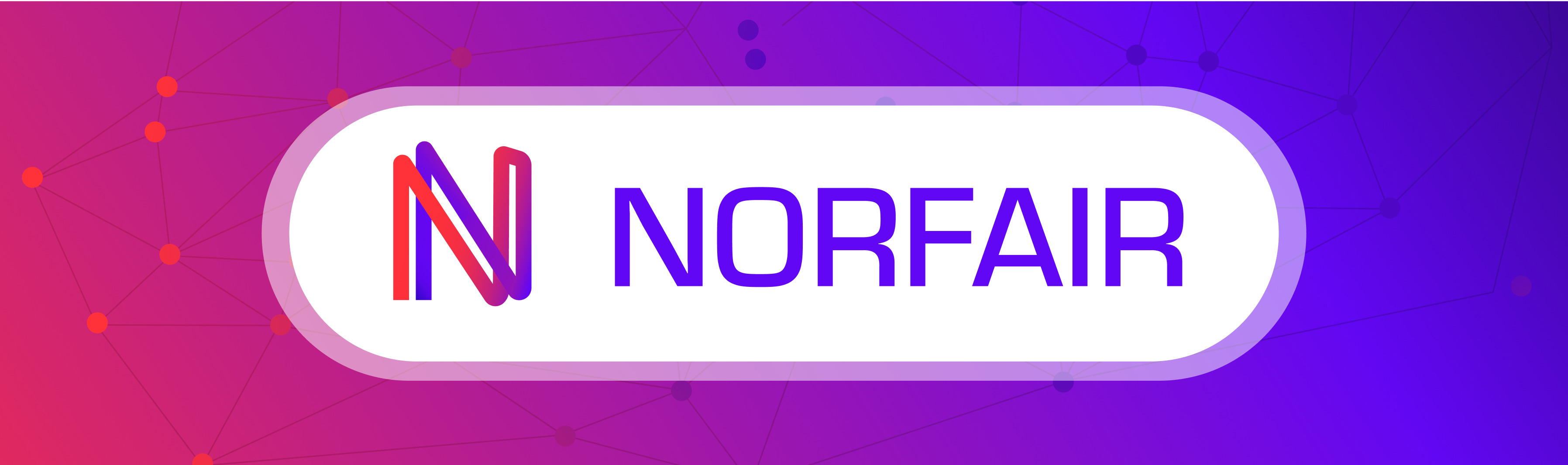
[](https://huggingface.co/spaces/tryolabs/norfair-demo)
[](https://colab.research.google.com/github/tryolabs/norfair/blob/master/demos/colab/colab_demo.ipynb)

[](https://pypi.org/project/norfair/)
[](https://tryolabs.github.io/norfair/)
[](https://github.com/tryolabs/norfair/projects/1)

[](https://zenodo.org/badge/latestdoi/276473370)
[](https://github.com/tryolabs/norfair/blob/master/LICENSE)
Norfair is a customizable lightweight Python library for real-time multi-object tracking.
Using Norfair, you can add tracking capabilities to any detector with just a few lines of code.
| Tracking players with moving camera | Tracking 3D objects |
| :---------------------------------------------------------------------------------------------------------------------: | :-----------------------------------------------------------------------------------------------------: |
| 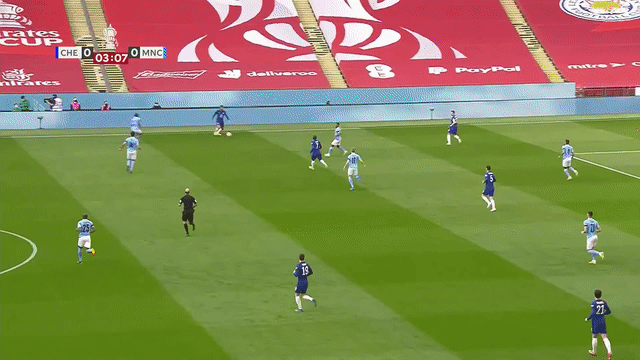 | 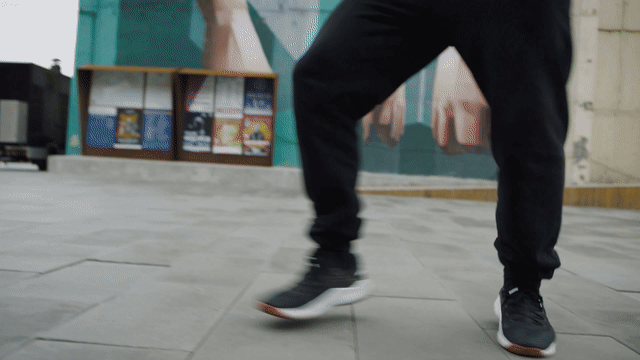 |
## Features
- Any detector expressing its detections as a series of `(x, y)` coordinates can be used with Norfair. This includes detectors performing tasks such as object or keypoint detection (see [examples](#examples--demos)).
- Modular. It can easily be inserted into complex video processing pipelines to add tracking to existing projects. At the same time, it is possible to build a video inference loop from scratch using just Norfair and a detector.
- Supports moving camera, re-identification with appearance embeddings, and n-dimensional object tracking (see [Advanced features](#advanced-features)).
- Norfair provides several predefined distance functions to compare tracked objects and detections. The distance functions can also be defined by the user, enabling the implementation of different tracking strategies.
- Fast. The only thing bounding inference speed will be the detection network feeding detections to Norfair.
Norfair is built, used and maintained by [Tryolabs](https://tryolabs.com).
## Installation
Norfair currently supports Python 3.7+.
For the minimal version, install as:
```bash
pip install norfair
```
To make Norfair install the dependencies to support more features, install as:
```bash
pip install norfair[video] # Adds several video helper features running on OpenCV
pip install norfair[metrics] # Supports running MOT metrics evaluation
pip install norfair[metrics,video] # Everything included
```
If the needed dependencies are already present in the system, installing the minimal version of Norfair is enough for enabling the extra features. This is particularly useful for embedded devices, where installing compiled dependencies can be difficult, but they can sometimes come preinstalled with the system.
## Documentation
[Getting started guide](https://tryolabs.github.io/norfair/latest/getting_started/).
[Official reference](https://tryolabs.github.io/norfair/latest/reference/).
## Examples & demos
[](https://huggingface.co/spaces/tryolabs/norfair-demo)
[](https://colab.research.google.com/github/tryolabs/norfair/blob/master/demos/colab/colab_demo.ipynb)
We provide several examples of how Norfair can be used to add tracking capabilities to different detectors, and also showcase more advanced features.
> Note: for ease of reproducibility, we provide Dockerfiles for all the demos. Even though Norfair does not need a GPU, the default configuration of most demos requires a GPU to be able to run the detectors. For this, make sure you install [NVIDIA Container Toolkit](https://docs.nvidia.com/datacenter/cloud-native/container-toolkit/install-guide.html) so that your GPU can be shared with Docker.
>
> It is possible to run several demos with a CPU, but you will have to modify the scripts or tinker with the installation of their dependencies.
### Adding tracking to different detectors
Most tracking demos are showcased with vehicles and pedestrians, but the detectors are generally trained with many more classes from the [COCO dataset](https://cocodataset.org/).
1. [YOLOv7](https://github.com/tryolabs/norfair/tree/master/demos/yolov7): tracking object centroids or bounding boxes.
2. [YOLOv5](https://github.com/tryolabs/norfair/tree/master/demos/yolov5): tracking object centroids or bounding boxes.
3. [YOLOv4](https://github.com/tryolabs/norfair/tree/master/demos/yolov4): tracking object centroids.
4. [Detectron2](https://github.com/tryolabs/norfair/tree/master/demos/detectron2): tracking object centroids.
5. [AlphaPose](https://github.com/tryolabs/norfair/tree/master/demos/alphapose): tracking human keypoints (pose estimation) and inserting Norfair into a complex existing pipeline using.
6. [OpenPose](https://github.com/tryolabs/norfair/tree/master/demos/openpose): tracking human keypoints.
7. [Tracking](https://github.com/tryolabs/norfair/tree/master/demos/yolopv2) objects with [YOLOPv2](https://github.com/CAIC-AD/YOLOPv2), a model for traffic object detection, drivable road area segmentation, and lane line detection.
### Advanced features
1. [Speed up pose estimation by extrapolating detections](https://github.com/tryolabs/norfair/tree/master/demos/openpose) using [OpenPose](https://github.com/CMU-Perceptual-Computing-Lab/openpose).
2. [Track both bounding boxes and human keypoints](https://github.com/tryolabs/norfair/tree/master/demos/keypoints_bounding_boxes) (multi-class), unifying the detections from a YOLO model and OpenPose.
3. [Re-identification (ReID)](https://github.com/tryolabs/norfair/tree/master/demos/reid) of tracked objects using appearance embeddings. This is a good starting point for scenarios with a lot of occlusion, in which the Kalman filter alone would struggle.
4. [Accurately track objects even if the camera is moving](https://github.com/tryolabs/norfair/tree/master/demos/camera_motion), by estimating camera motion potentially accounting for pan, tilt, rotation, movement in any direction, and zoom.
5. [Track points in 3D](https://github.com/tryolabs/norfair/tree/master/demos/3d_track), using [MediaPipe Objectron](https://google.github.io/mediapipe/solutions/objectron.html).
6. [Tracking of small objects](https://github.com/tryolabs/norfair/tree/master/demos/sahi), using [SAHI: Slicing Aided Hyper Inference](https://github.com/obss/sahi).
### ROS integration
To make it even easier to use Norfair in robotics projects, we now offer a version that integrates with the Robotic Operating System (ROS).
We present a [ROS package](https://github.com/tryolabs/norfair-ros) and a [fully functional environment](https://github.com/tryolabs/norfair-ros-dev) running on Docker to do the first steps with this package and start your first application easier.
### Benchmarking and profiling
1. [Kalman filter and distance function profiling](https://github.com/tryolabs/norfair/tree/master/demos/profiling) using [TRT pose estimator](https://github.com/NVIDIA-AI-IOT/trt_pose).
2. Computation of [MOT17](https://motchallenge.net/data/MOT17/) scores using [motmetrics4norfair](https://github.com/tryolabs/norfair/tree/master/demos/motmetrics4norfair).
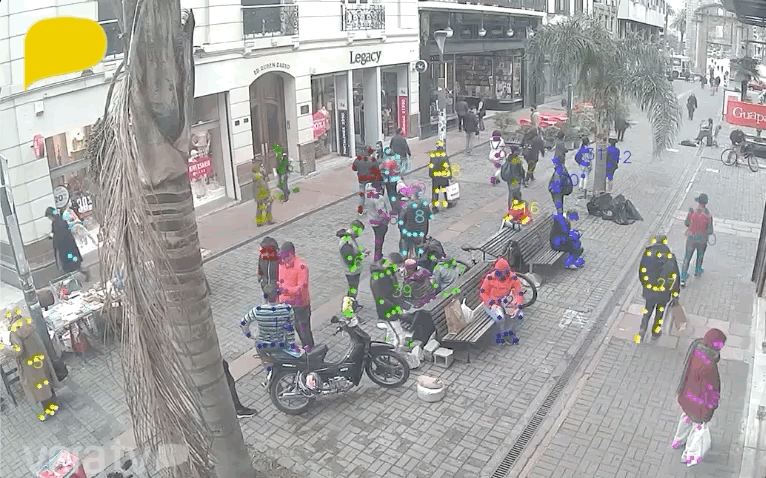
## How it works
Norfair works by estimating the future position of each point based on its past positions. It then tries to match these estimated positions with newly detected points provided by the detector. For this matching to occur, Norfair can rely on any distance function. There are some predefined distances already integrated in Norfair, and the users can also define their own custom distances. Therefore, each object tracker can be made as simple or as complex as needed.
As an example we use [Detectron2](https://github.com/facebookresearch/detectron2) to get the single point detections to use with this distance function. We just use the centroids of the bounding boxes it produces around cars as our detections, and get the following results.
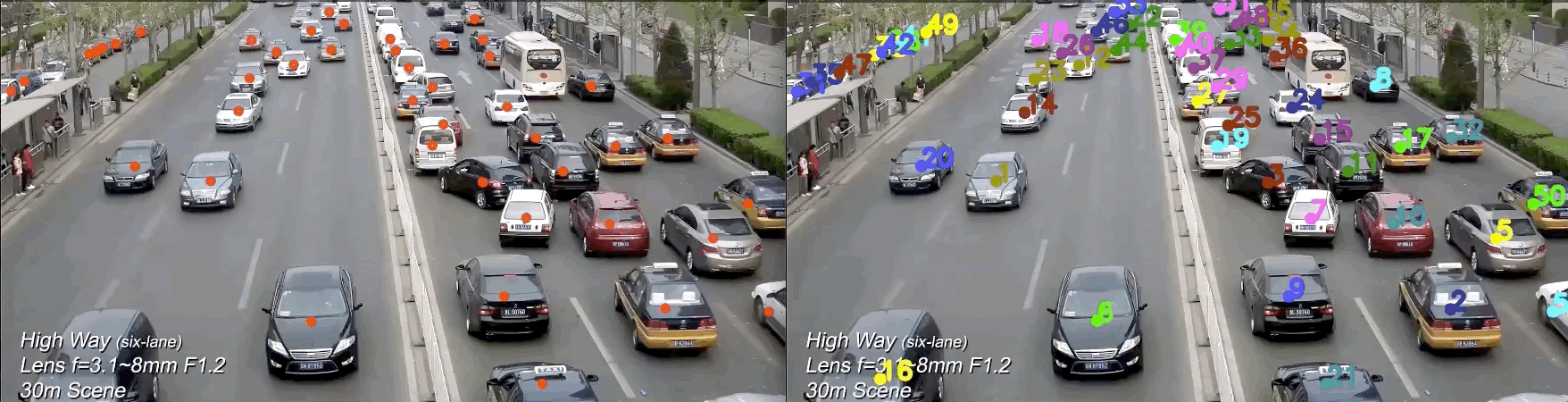
On the left you can see the points we get from Detectron2, and on the right how Norfair tracks them assigning a unique identifier through time. Even a straightforward distance function like this one can work when the tracking needed is simple.
Norfair also provides several useful tools for creating a video inference loop. Here is what the full code for creating the previous example looks like, including the code needed to set up Detectron2:
```python
import cv2
import numpy as np
from detectron2.config import get_cfg
from detectron2.engine import DefaultPredictor
from norfair import Detection, Tracker, Video, draw_tracked_objects
# Set up Detectron2 object detector
cfg = get_cfg()
cfg.merge_from_file("demos/faster_rcnn_R_50_FPN_3x.yaml")
cfg.MODEL.ROI_HEADS.SCORE_THRESH_TEST = 0.5
cfg.MODEL.WEIGHTS = "detectron2://COCO-InstanceSegmentation/mask_rcnn_R_50_FPN_3x/137849600/model_final_f10217.pkl"
detector = DefaultPredictor(cfg)
# Norfair
video = Video(input_path="video.mp4")
tracker = Tracker(distance_function="euclidean", distance_threshold=20)
for frame in video:
detections = detector(cv2.cvtColor(frame, cv2.COLOR_BGR2RGB))
detections = [Detection(p) for p in detections['instances'].pred_boxes.get_centers().cpu().numpy()]
tracked_objects = tracker.update(detections=detections)
draw_tracked_objects(frame, tracked_objects)
video.write(frame)
```
The video and drawing tools use OpenCV frames, so they are compatible with most Python video code available online. The point tracking is based on [SORT](https://arxiv.org/abs/1602.00763) generalized to detections consisting of a dynamically changing number of points per detection.
## Motivation
Trying out the latest state-of-the-art detectors normally requires running repositories that weren't intended to be easy to use. These tend to be repositories associated with a research paper describing a novel new way of doing detection, and they are therefore intended to be run as a one-off evaluation script to get some result metric to publish on a particular research paper. This explains why they tend to not be easy to run as inference scripts, or why extracting the core model to use in another standalone script isn't always trivial.
Norfair was born out of the need to quickly add a simple layer of tracking over a wide range of newly released SOTA detectors. It was designed to seamlessly be plugged into a complex, highly coupled code base, with minimum effort. Norfair provides a series of modular but compatible tools, which you can pick and choose to use in your project.
## Comparison to other trackers
Norfair's contribution to Python's object tracker library repertoire is its ability to work with any object detector by being able to work with a variable number of points per detection, and the ability for the user to heavily customize the tracker by creating their own distance function.
If you are looking for a tracker, here are some other projects worth noting:
- [**OpenCV**](https://opencv.org) includes several tracking solutions like [KCF Tracker](https://docs.opencv.org/3.4/d2/dff/classcv_1_1TrackerKCF.html) and [MedianFlow Tracker](https://docs.opencv.org/3.4/d7/d86/classcv_1_1TrackerMedianFlow.html) which are run by making the user select a part of the frame to track, and then letting the tracker follow that area. They tend not to be run on top of a detector and are not very robust.
- [**dlib**](http://dlib.net) includes a correlation single object tracker. You have to create your own multiple object tracker on top of it yourself if you want to track multiple objects with it.
- [**AlphaPose**](https://github.com/MVIG-SJTU/AlphaPose) just released a new version of their human pose tracker. This tracker is tightly integrated into their code base, and to the task of tracking human poses.
- [**SORT**](https://github.com/abewley/sort) and [**Deep SORT**](https://github.com/nwojke/deep_sort) are similar to this repo in that they use Kalman filters (and a deep embedding for Deep SORT), but they are hardcoded to a fixed distance function and to tracking boxes. Norfair also adds some filtering when matching tracked objects with detections, and changes the Hungarian Algorithm for its own distance minimizer. Both these repos are also released under the GPL license, which might be an issue for some individuals or companies because the source code of derivative works needs to be published.
## Benchmarks
[MOT17](https://motchallenge.net/data/MOT17/) and [MOT20](https://motchallenge.net/data/MOT17/) results obtained using [motmetrics4norfair](https://github.com/tryolabs/norfair/tree/master/demos/motmetrics4norfair) demo script on the `train` split. We used detections obtained with [ByteTrack's](https://github.com/ifzhang/ByteTrack) YOLOX object detection model.
| MOT17 Train | IDF1 IDP IDR | Rcll | Prcn | MOTA MOTP |
| :---------: | :---------------: | :---: | :---: | :---------: |
| MOT17-02 | 61.3% 63.6% 59.0% | 86.8% | 93.5% | 79.9% 14.8% |
| MOT17-04 | 93.3% 93.6% 93.0% | 98.6% | 99.3% | 97.9% 07.9% |
| MOT17-05 | 77.8% 77.7% 77.8% | 85.9% | 85.8% | 71.2% 14.7% |
| MOT17-09 | 65.0% 67.4% 62.9% | 90.3% | 96.8% | 86.8% 12.2% |
| MOT17-10 | 70.2% 72.5% 68.1% | 87.3% | 93.0% | 80.1% 18.7% |
| MOT17-11 | 80.2% 80.5% 80.0% | 93.0% | 93.6% | 86.4% 11.3% |
| MOT17-13 | 79.0% 79.6% 78.4% | 90.6% | 92.0% | 82.4% 16.6% |
| OVERALL | 80.6% 81.8% 79.6% | 92.9% | 95.5% | 88.1% 11.9% |
| MOT20 Train | IDF1 IDP IDR | Rcll | Prcn | MOTA MOTP |
| :---------: | :---------------: | :---: | :---: | :---------: |
| MOT20-01 | 85.9% 88.1% 83.8% | 93.4% | 98.2% | 91.5% 12.6% |
| MOT20-02 | 72.8% 74.6% 71.0% | 93.2% | 97.9% | 91.0% 12.7% |
| MOT20-03 | 93.0% 94.1% 92.0% | 96.1% | 98.3% | 94.4% 13.7% |
| MOT20-05 | 87.9% 88.9% 87.0% | 96.0% | 98.1% | 94.1% 13.0% |
| OVERALL | 87.3% 88.4% 86.2% | 95.6% | 98.1% | 93.7% 13.2% |
## Commercial support
Tryolabs can provide commercial support, implement new features in Norfair or build video analytics tools for solving your challenging problems. Norfair powers several video analytics applications, such as the [face mask detection](https://tryolabs.com/blog/2020/07/09/face-mask-detection-in-street-camera-video-streams-using-ai-behind-the-curtain/) tool.
If you are interested, please [contact us](mailto:hello@tryolabs.com).
## Citing Norfair
For citations in academic publications, please export your desired citation format (BibTeX or other) from [Zenodo](https://doi.org/10.5281/zenodo.5146253).
## License
Copyright © 2022, [Tryolabs](https://tryolabs.com). Released under the [BSD 3-Clause](https://github.com/tryolabs/norfair/blob/master/LICENSE).
%package -n python3-norfair
Summary: Lightweight Python library for adding real-time multi-object tracking to any detector.
Provides: python-norfair
BuildRequires: python3-devel
BuildRequires: python3-setuptools
BuildRequires: python3-pip
%description -n python3-norfair
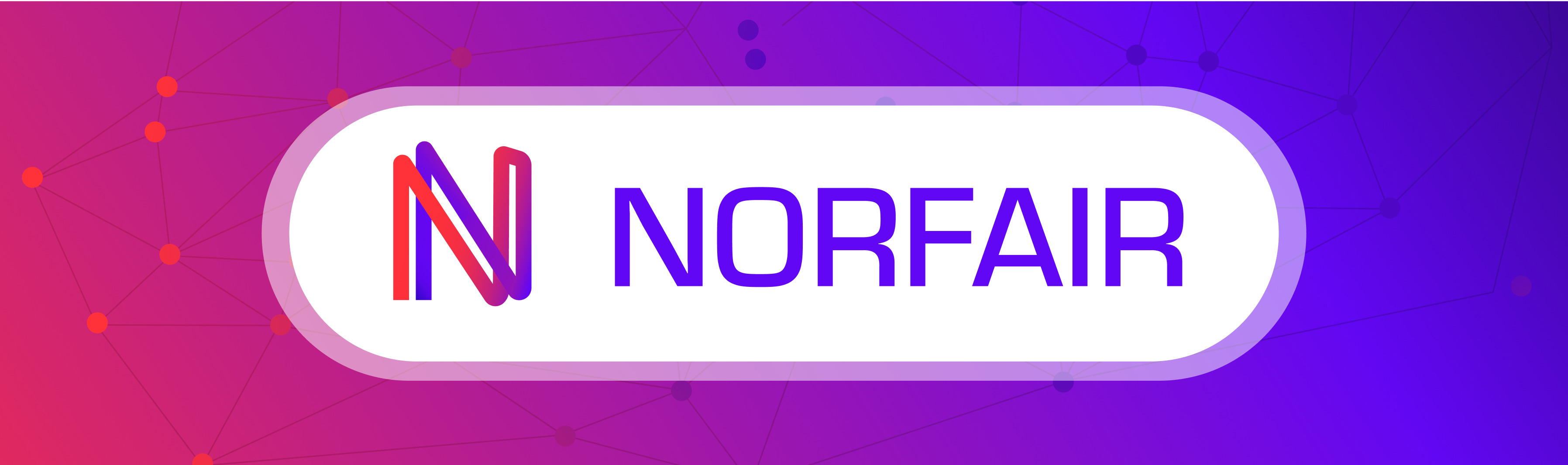
[](https://huggingface.co/spaces/tryolabs/norfair-demo)
[](https://colab.research.google.com/github/tryolabs/norfair/blob/master/demos/colab/colab_demo.ipynb)

[](https://pypi.org/project/norfair/)
[](https://tryolabs.github.io/norfair/)
[](https://github.com/tryolabs/norfair/projects/1)

[](https://zenodo.org/badge/latestdoi/276473370)
[](https://github.com/tryolabs/norfair/blob/master/LICENSE)
Norfair is a customizable lightweight Python library for real-time multi-object tracking.
Using Norfair, you can add tracking capabilities to any detector with just a few lines of code.
| Tracking players with moving camera | Tracking 3D objects |
| :---------------------------------------------------------------------------------------------------------------------: | :-----------------------------------------------------------------------------------------------------: |
| 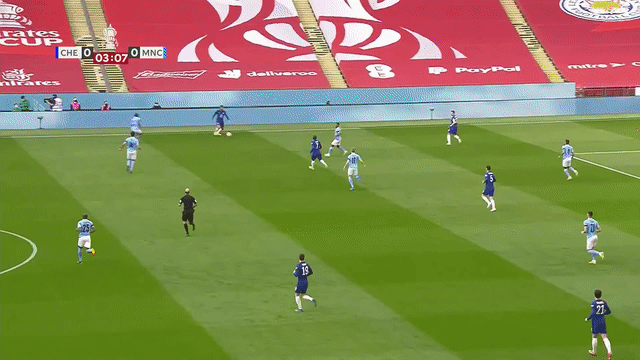 | 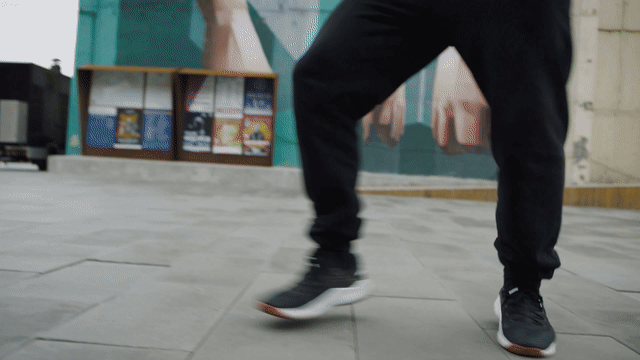 |
## Features
- Any detector expressing its detections as a series of `(x, y)` coordinates can be used with Norfair. This includes detectors performing tasks such as object or keypoint detection (see [examples](#examples--demos)).
- Modular. It can easily be inserted into complex video processing pipelines to add tracking to existing projects. At the same time, it is possible to build a video inference loop from scratch using just Norfair and a detector.
- Supports moving camera, re-identification with appearance embeddings, and n-dimensional object tracking (see [Advanced features](#advanced-features)).
- Norfair provides several predefined distance functions to compare tracked objects and detections. The distance functions can also be defined by the user, enabling the implementation of different tracking strategies.
- Fast. The only thing bounding inference speed will be the detection network feeding detections to Norfair.
Norfair is built, used and maintained by [Tryolabs](https://tryolabs.com).
## Installation
Norfair currently supports Python 3.7+.
For the minimal version, install as:
```bash
pip install norfair
```
To make Norfair install the dependencies to support more features, install as:
```bash
pip install norfair[video] # Adds several video helper features running on OpenCV
pip install norfair[metrics] # Supports running MOT metrics evaluation
pip install norfair[metrics,video] # Everything included
```
If the needed dependencies are already present in the system, installing the minimal version of Norfair is enough for enabling the extra features. This is particularly useful for embedded devices, where installing compiled dependencies can be difficult, but they can sometimes come preinstalled with the system.
## Documentation
[Getting started guide](https://tryolabs.github.io/norfair/latest/getting_started/).
[Official reference](https://tryolabs.github.io/norfair/latest/reference/).
## Examples & demos
[](https://huggingface.co/spaces/tryolabs/norfair-demo)
[](https://colab.research.google.com/github/tryolabs/norfair/blob/master/demos/colab/colab_demo.ipynb)
We provide several examples of how Norfair can be used to add tracking capabilities to different detectors, and also showcase more advanced features.
> Note: for ease of reproducibility, we provide Dockerfiles for all the demos. Even though Norfair does not need a GPU, the default configuration of most demos requires a GPU to be able to run the detectors. For this, make sure you install [NVIDIA Container Toolkit](https://docs.nvidia.com/datacenter/cloud-native/container-toolkit/install-guide.html) so that your GPU can be shared with Docker.
>
> It is possible to run several demos with a CPU, but you will have to modify the scripts or tinker with the installation of their dependencies.
### Adding tracking to different detectors
Most tracking demos are showcased with vehicles and pedestrians, but the detectors are generally trained with many more classes from the [COCO dataset](https://cocodataset.org/).
1. [YOLOv7](https://github.com/tryolabs/norfair/tree/master/demos/yolov7): tracking object centroids or bounding boxes.
2. [YOLOv5](https://github.com/tryolabs/norfair/tree/master/demos/yolov5): tracking object centroids or bounding boxes.
3. [YOLOv4](https://github.com/tryolabs/norfair/tree/master/demos/yolov4): tracking object centroids.
4. [Detectron2](https://github.com/tryolabs/norfair/tree/master/demos/detectron2): tracking object centroids.
5. [AlphaPose](https://github.com/tryolabs/norfair/tree/master/demos/alphapose): tracking human keypoints (pose estimation) and inserting Norfair into a complex existing pipeline using.
6. [OpenPose](https://github.com/tryolabs/norfair/tree/master/demos/openpose): tracking human keypoints.
7. [Tracking](https://github.com/tryolabs/norfair/tree/master/demos/yolopv2) objects with [YOLOPv2](https://github.com/CAIC-AD/YOLOPv2), a model for traffic object detection, drivable road area segmentation, and lane line detection.
### Advanced features
1. [Speed up pose estimation by extrapolating detections](https://github.com/tryolabs/norfair/tree/master/demos/openpose) using [OpenPose](https://github.com/CMU-Perceptual-Computing-Lab/openpose).
2. [Track both bounding boxes and human keypoints](https://github.com/tryolabs/norfair/tree/master/demos/keypoints_bounding_boxes) (multi-class), unifying the detections from a YOLO model and OpenPose.
3. [Re-identification (ReID)](https://github.com/tryolabs/norfair/tree/master/demos/reid) of tracked objects using appearance embeddings. This is a good starting point for scenarios with a lot of occlusion, in which the Kalman filter alone would struggle.
4. [Accurately track objects even if the camera is moving](https://github.com/tryolabs/norfair/tree/master/demos/camera_motion), by estimating camera motion potentially accounting for pan, tilt, rotation, movement in any direction, and zoom.
5. [Track points in 3D](https://github.com/tryolabs/norfair/tree/master/demos/3d_track), using [MediaPipe Objectron](https://google.github.io/mediapipe/solutions/objectron.html).
6. [Tracking of small objects](https://github.com/tryolabs/norfair/tree/master/demos/sahi), using [SAHI: Slicing Aided Hyper Inference](https://github.com/obss/sahi).
### ROS integration
To make it even easier to use Norfair in robotics projects, we now offer a version that integrates with the Robotic Operating System (ROS).
We present a [ROS package](https://github.com/tryolabs/norfair-ros) and a [fully functional environment](https://github.com/tryolabs/norfair-ros-dev) running on Docker to do the first steps with this package and start your first application easier.
### Benchmarking and profiling
1. [Kalman filter and distance function profiling](https://github.com/tryolabs/norfair/tree/master/demos/profiling) using [TRT pose estimator](https://github.com/NVIDIA-AI-IOT/trt_pose).
2. Computation of [MOT17](https://motchallenge.net/data/MOT17/) scores using [motmetrics4norfair](https://github.com/tryolabs/norfair/tree/master/demos/motmetrics4norfair).
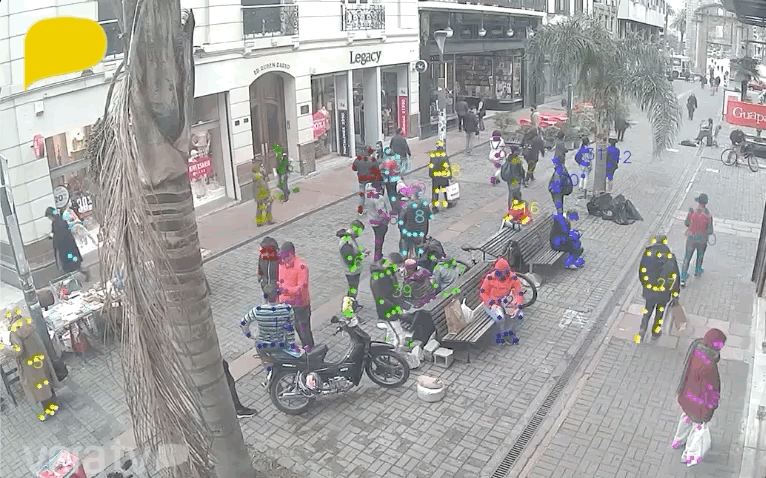
## How it works
Norfair works by estimating the future position of each point based on its past positions. It then tries to match these estimated positions with newly detected points provided by the detector. For this matching to occur, Norfair can rely on any distance function. There are some predefined distances already integrated in Norfair, and the users can also define their own custom distances. Therefore, each object tracker can be made as simple or as complex as needed.
As an example we use [Detectron2](https://github.com/facebookresearch/detectron2) to get the single point detections to use with this distance function. We just use the centroids of the bounding boxes it produces around cars as our detections, and get the following results.
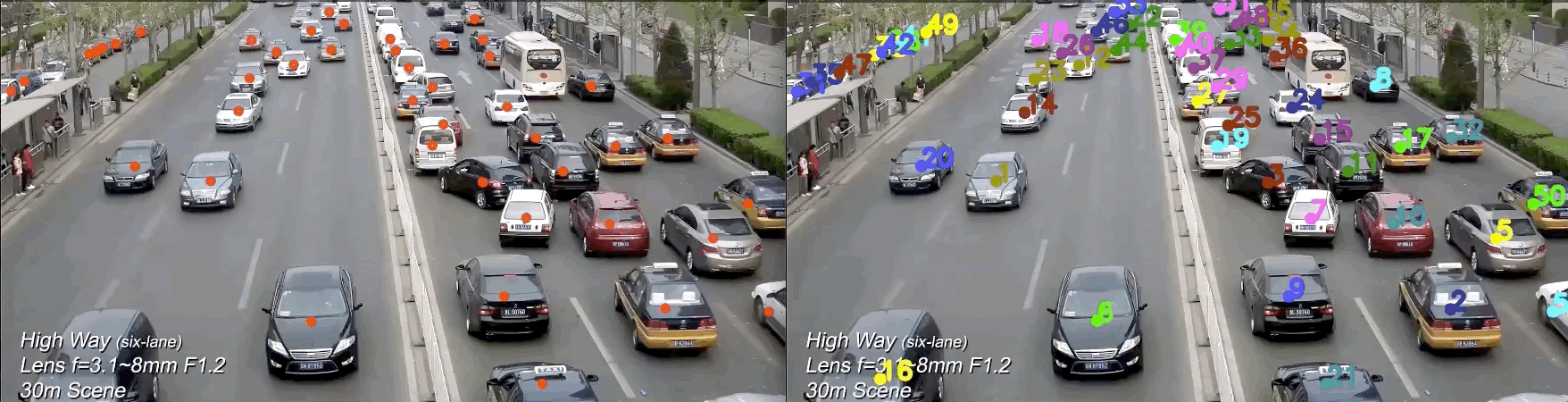
On the left you can see the points we get from Detectron2, and on the right how Norfair tracks them assigning a unique identifier through time. Even a straightforward distance function like this one can work when the tracking needed is simple.
Norfair also provides several useful tools for creating a video inference loop. Here is what the full code for creating the previous example looks like, including the code needed to set up Detectron2:
```python
import cv2
import numpy as np
from detectron2.config import get_cfg
from detectron2.engine import DefaultPredictor
from norfair import Detection, Tracker, Video, draw_tracked_objects
# Set up Detectron2 object detector
cfg = get_cfg()
cfg.merge_from_file("demos/faster_rcnn_R_50_FPN_3x.yaml")
cfg.MODEL.ROI_HEADS.SCORE_THRESH_TEST = 0.5
cfg.MODEL.WEIGHTS = "detectron2://COCO-InstanceSegmentation/mask_rcnn_R_50_FPN_3x/137849600/model_final_f10217.pkl"
detector = DefaultPredictor(cfg)
# Norfair
video = Video(input_path="video.mp4")
tracker = Tracker(distance_function="euclidean", distance_threshold=20)
for frame in video:
detections = detector(cv2.cvtColor(frame, cv2.COLOR_BGR2RGB))
detections = [Detection(p) for p in detections['instances'].pred_boxes.get_centers().cpu().numpy()]
tracked_objects = tracker.update(detections=detections)
draw_tracked_objects(frame, tracked_objects)
video.write(frame)
```
The video and drawing tools use OpenCV frames, so they are compatible with most Python video code available online. The point tracking is based on [SORT](https://arxiv.org/abs/1602.00763) generalized to detections consisting of a dynamically changing number of points per detection.
## Motivation
Trying out the latest state-of-the-art detectors normally requires running repositories that weren't intended to be easy to use. These tend to be repositories associated with a research paper describing a novel new way of doing detection, and they are therefore intended to be run as a one-off evaluation script to get some result metric to publish on a particular research paper. This explains why they tend to not be easy to run as inference scripts, or why extracting the core model to use in another standalone script isn't always trivial.
Norfair was born out of the need to quickly add a simple layer of tracking over a wide range of newly released SOTA detectors. It was designed to seamlessly be plugged into a complex, highly coupled code base, with minimum effort. Norfair provides a series of modular but compatible tools, which you can pick and choose to use in your project.
## Comparison to other trackers
Norfair's contribution to Python's object tracker library repertoire is its ability to work with any object detector by being able to work with a variable number of points per detection, and the ability for the user to heavily customize the tracker by creating their own distance function.
If you are looking for a tracker, here are some other projects worth noting:
- [**OpenCV**](https://opencv.org) includes several tracking solutions like [KCF Tracker](https://docs.opencv.org/3.4/d2/dff/classcv_1_1TrackerKCF.html) and [MedianFlow Tracker](https://docs.opencv.org/3.4/d7/d86/classcv_1_1TrackerMedianFlow.html) which are run by making the user select a part of the frame to track, and then letting the tracker follow that area. They tend not to be run on top of a detector and are not very robust.
- [**dlib**](http://dlib.net) includes a correlation single object tracker. You have to create your own multiple object tracker on top of it yourself if you want to track multiple objects with it.
- [**AlphaPose**](https://github.com/MVIG-SJTU/AlphaPose) just released a new version of their human pose tracker. This tracker is tightly integrated into their code base, and to the task of tracking human poses.
- [**SORT**](https://github.com/abewley/sort) and [**Deep SORT**](https://github.com/nwojke/deep_sort) are similar to this repo in that they use Kalman filters (and a deep embedding for Deep SORT), but they are hardcoded to a fixed distance function and to tracking boxes. Norfair also adds some filtering when matching tracked objects with detections, and changes the Hungarian Algorithm for its own distance minimizer. Both these repos are also released under the GPL license, which might be an issue for some individuals or companies because the source code of derivative works needs to be published.
## Benchmarks
[MOT17](https://motchallenge.net/data/MOT17/) and [MOT20](https://motchallenge.net/data/MOT17/) results obtained using [motmetrics4norfair](https://github.com/tryolabs/norfair/tree/master/demos/motmetrics4norfair) demo script on the `train` split. We used detections obtained with [ByteTrack's](https://github.com/ifzhang/ByteTrack) YOLOX object detection model.
| MOT17 Train | IDF1 IDP IDR | Rcll | Prcn | MOTA MOTP |
| :---------: | :---------------: | :---: | :---: | :---------: |
| MOT17-02 | 61.3% 63.6% 59.0% | 86.8% | 93.5% | 79.9% 14.8% |
| MOT17-04 | 93.3% 93.6% 93.0% | 98.6% | 99.3% | 97.9% 07.9% |
| MOT17-05 | 77.8% 77.7% 77.8% | 85.9% | 85.8% | 71.2% 14.7% |
| MOT17-09 | 65.0% 67.4% 62.9% | 90.3% | 96.8% | 86.8% 12.2% |
| MOT17-10 | 70.2% 72.5% 68.1% | 87.3% | 93.0% | 80.1% 18.7% |
| MOT17-11 | 80.2% 80.5% 80.0% | 93.0% | 93.6% | 86.4% 11.3% |
| MOT17-13 | 79.0% 79.6% 78.4% | 90.6% | 92.0% | 82.4% 16.6% |
| OVERALL | 80.6% 81.8% 79.6% | 92.9% | 95.5% | 88.1% 11.9% |
| MOT20 Train | IDF1 IDP IDR | Rcll | Prcn | MOTA MOTP |
| :---------: | :---------------: | :---: | :---: | :---------: |
| MOT20-01 | 85.9% 88.1% 83.8% | 93.4% | 98.2% | 91.5% 12.6% |
| MOT20-02 | 72.8% 74.6% 71.0% | 93.2% | 97.9% | 91.0% 12.7% |
| MOT20-03 | 93.0% 94.1% 92.0% | 96.1% | 98.3% | 94.4% 13.7% |
| MOT20-05 | 87.9% 88.9% 87.0% | 96.0% | 98.1% | 94.1% 13.0% |
| OVERALL | 87.3% 88.4% 86.2% | 95.6% | 98.1% | 93.7% 13.2% |
## Commercial support
Tryolabs can provide commercial support, implement new features in Norfair or build video analytics tools for solving your challenging problems. Norfair powers several video analytics applications, such as the [face mask detection](https://tryolabs.com/blog/2020/07/09/face-mask-detection-in-street-camera-video-streams-using-ai-behind-the-curtain/) tool.
If you are interested, please [contact us](mailto:hello@tryolabs.com).
## Citing Norfair
For citations in academic publications, please export your desired citation format (BibTeX or other) from [Zenodo](https://doi.org/10.5281/zenodo.5146253).
## License
Copyright © 2022, [Tryolabs](https://tryolabs.com). Released under the [BSD 3-Clause](https://github.com/tryolabs/norfair/blob/master/LICENSE).
%package help
Summary: Development documents and examples for norfair
Provides: python3-norfair-doc
%description help
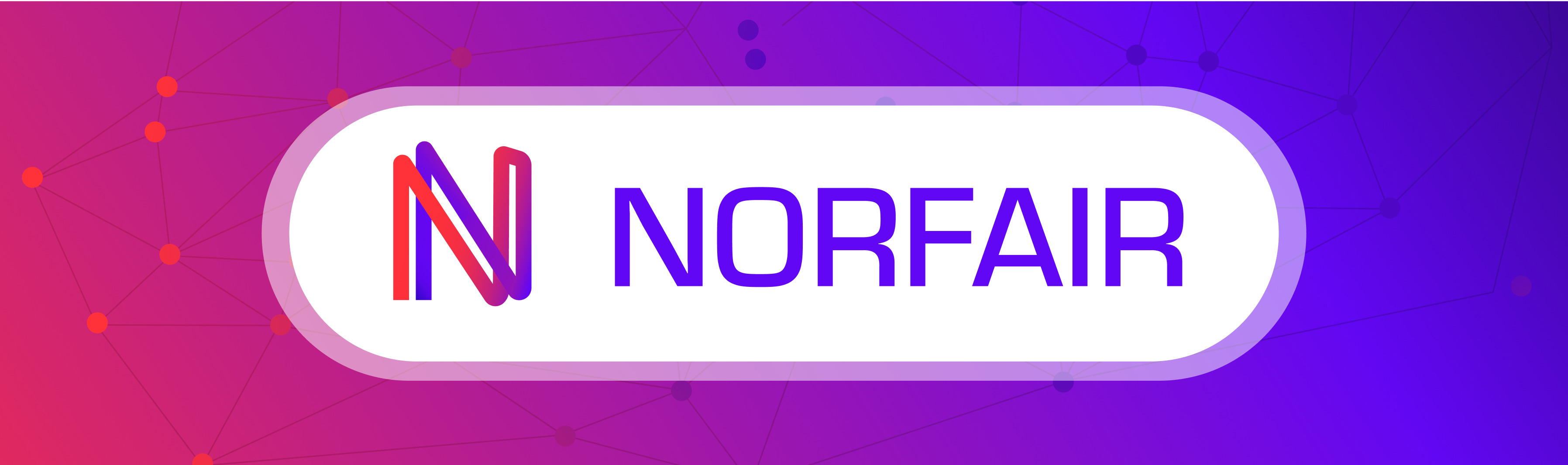
[](https://huggingface.co/spaces/tryolabs/norfair-demo)
[](https://colab.research.google.com/github/tryolabs/norfair/blob/master/demos/colab/colab_demo.ipynb)

[](https://pypi.org/project/norfair/)
[](https://tryolabs.github.io/norfair/)
[](https://github.com/tryolabs/norfair/projects/1)

[](https://zenodo.org/badge/latestdoi/276473370)
[](https://github.com/tryolabs/norfair/blob/master/LICENSE)
Norfair is a customizable lightweight Python library for real-time multi-object tracking.
Using Norfair, you can add tracking capabilities to any detector with just a few lines of code.
| Tracking players with moving camera | Tracking 3D objects |
| :---------------------------------------------------------------------------------------------------------------------: | :-----------------------------------------------------------------------------------------------------: |
| 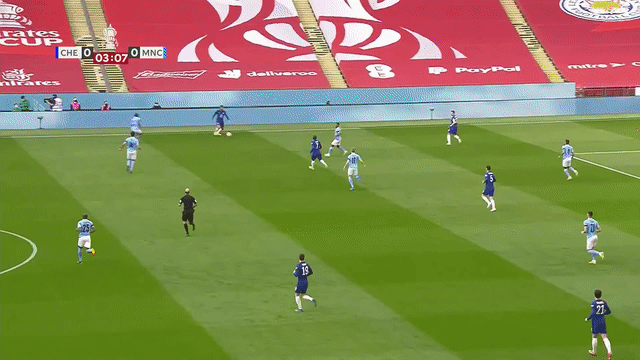 | 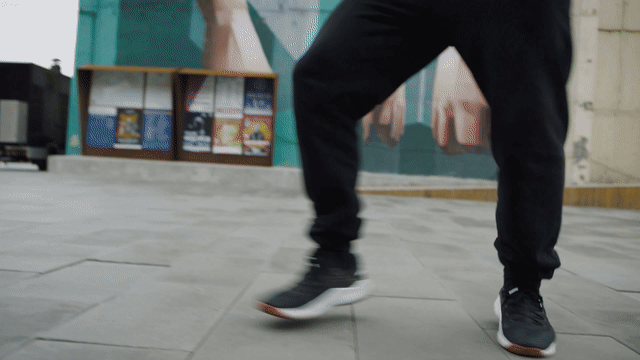 |
## Features
- Any detector expressing its detections as a series of `(x, y)` coordinates can be used with Norfair. This includes detectors performing tasks such as object or keypoint detection (see [examples](#examples--demos)).
- Modular. It can easily be inserted into complex video processing pipelines to add tracking to existing projects. At the same time, it is possible to build a video inference loop from scratch using just Norfair and a detector.
- Supports moving camera, re-identification with appearance embeddings, and n-dimensional object tracking (see [Advanced features](#advanced-features)).
- Norfair provides several predefined distance functions to compare tracked objects and detections. The distance functions can also be defined by the user, enabling the implementation of different tracking strategies.
- Fast. The only thing bounding inference speed will be the detection network feeding detections to Norfair.
Norfair is built, used and maintained by [Tryolabs](https://tryolabs.com).
## Installation
Norfair currently supports Python 3.7+.
For the minimal version, install as:
```bash
pip install norfair
```
To make Norfair install the dependencies to support more features, install as:
```bash
pip install norfair[video] # Adds several video helper features running on OpenCV
pip install norfair[metrics] # Supports running MOT metrics evaluation
pip install norfair[metrics,video] # Everything included
```
If the needed dependencies are already present in the system, installing the minimal version of Norfair is enough for enabling the extra features. This is particularly useful for embedded devices, where installing compiled dependencies can be difficult, but they can sometimes come preinstalled with the system.
## Documentation
[Getting started guide](https://tryolabs.github.io/norfair/latest/getting_started/).
[Official reference](https://tryolabs.github.io/norfair/latest/reference/).
## Examples & demos
[](https://huggingface.co/spaces/tryolabs/norfair-demo)
[](https://colab.research.google.com/github/tryolabs/norfair/blob/master/demos/colab/colab_demo.ipynb)
We provide several examples of how Norfair can be used to add tracking capabilities to different detectors, and also showcase more advanced features.
> Note: for ease of reproducibility, we provide Dockerfiles for all the demos. Even though Norfair does not need a GPU, the default configuration of most demos requires a GPU to be able to run the detectors. For this, make sure you install [NVIDIA Container Toolkit](https://docs.nvidia.com/datacenter/cloud-native/container-toolkit/install-guide.html) so that your GPU can be shared with Docker.
>
> It is possible to run several demos with a CPU, but you will have to modify the scripts or tinker with the installation of their dependencies.
### Adding tracking to different detectors
Most tracking demos are showcased with vehicles and pedestrians, but the detectors are generally trained with many more classes from the [COCO dataset](https://cocodataset.org/).
1. [YOLOv7](https://github.com/tryolabs/norfair/tree/master/demos/yolov7): tracking object centroids or bounding boxes.
2. [YOLOv5](https://github.com/tryolabs/norfair/tree/master/demos/yolov5): tracking object centroids or bounding boxes.
3. [YOLOv4](https://github.com/tryolabs/norfair/tree/master/demos/yolov4): tracking object centroids.
4. [Detectron2](https://github.com/tryolabs/norfair/tree/master/demos/detectron2): tracking object centroids.
5. [AlphaPose](https://github.com/tryolabs/norfair/tree/master/demos/alphapose): tracking human keypoints (pose estimation) and inserting Norfair into a complex existing pipeline using.
6. [OpenPose](https://github.com/tryolabs/norfair/tree/master/demos/openpose): tracking human keypoints.
7. [Tracking](https://github.com/tryolabs/norfair/tree/master/demos/yolopv2) objects with [YOLOPv2](https://github.com/CAIC-AD/YOLOPv2), a model for traffic object detection, drivable road area segmentation, and lane line detection.
### Advanced features
1. [Speed up pose estimation by extrapolating detections](https://github.com/tryolabs/norfair/tree/master/demos/openpose) using [OpenPose](https://github.com/CMU-Perceptual-Computing-Lab/openpose).
2. [Track both bounding boxes and human keypoints](https://github.com/tryolabs/norfair/tree/master/demos/keypoints_bounding_boxes) (multi-class), unifying the detections from a YOLO model and OpenPose.
3. [Re-identification (ReID)](https://github.com/tryolabs/norfair/tree/master/demos/reid) of tracked objects using appearance embeddings. This is a good starting point for scenarios with a lot of occlusion, in which the Kalman filter alone would struggle.
4. [Accurately track objects even if the camera is moving](https://github.com/tryolabs/norfair/tree/master/demos/camera_motion), by estimating camera motion potentially accounting for pan, tilt, rotation, movement in any direction, and zoom.
5. [Track points in 3D](https://github.com/tryolabs/norfair/tree/master/demos/3d_track), using [MediaPipe Objectron](https://google.github.io/mediapipe/solutions/objectron.html).
6. [Tracking of small objects](https://github.com/tryolabs/norfair/tree/master/demos/sahi), using [SAHI: Slicing Aided Hyper Inference](https://github.com/obss/sahi).
### ROS integration
To make it even easier to use Norfair in robotics projects, we now offer a version that integrates with the Robotic Operating System (ROS).
We present a [ROS package](https://github.com/tryolabs/norfair-ros) and a [fully functional environment](https://github.com/tryolabs/norfair-ros-dev) running on Docker to do the first steps with this package and start your first application easier.
### Benchmarking and profiling
1. [Kalman filter and distance function profiling](https://github.com/tryolabs/norfair/tree/master/demos/profiling) using [TRT pose estimator](https://github.com/NVIDIA-AI-IOT/trt_pose).
2. Computation of [MOT17](https://motchallenge.net/data/MOT17/) scores using [motmetrics4norfair](https://github.com/tryolabs/norfair/tree/master/demos/motmetrics4norfair).
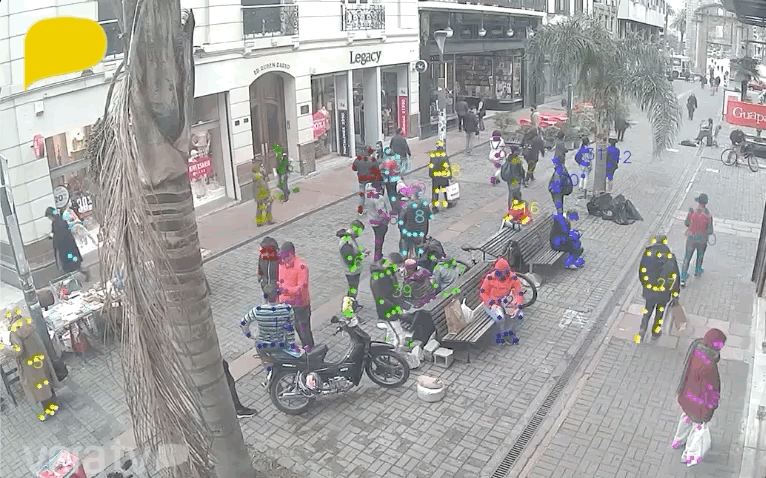
## How it works
Norfair works by estimating the future position of each point based on its past positions. It then tries to match these estimated positions with newly detected points provided by the detector. For this matching to occur, Norfair can rely on any distance function. There are some predefined distances already integrated in Norfair, and the users can also define their own custom distances. Therefore, each object tracker can be made as simple or as complex as needed.
As an example we use [Detectron2](https://github.com/facebookresearch/detectron2) to get the single point detections to use with this distance function. We just use the centroids of the bounding boxes it produces around cars as our detections, and get the following results.
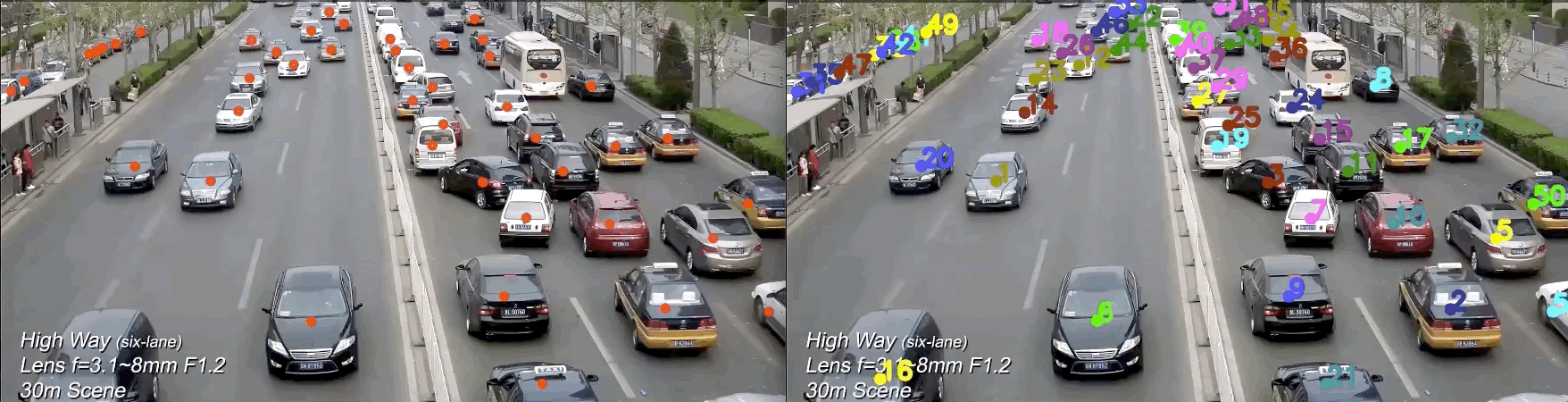
On the left you can see the points we get from Detectron2, and on the right how Norfair tracks them assigning a unique identifier through time. Even a straightforward distance function like this one can work when the tracking needed is simple.
Norfair also provides several useful tools for creating a video inference loop. Here is what the full code for creating the previous example looks like, including the code needed to set up Detectron2:
```python
import cv2
import numpy as np
from detectron2.config import get_cfg
from detectron2.engine import DefaultPredictor
from norfair import Detection, Tracker, Video, draw_tracked_objects
# Set up Detectron2 object detector
cfg = get_cfg()
cfg.merge_from_file("demos/faster_rcnn_R_50_FPN_3x.yaml")
cfg.MODEL.ROI_HEADS.SCORE_THRESH_TEST = 0.5
cfg.MODEL.WEIGHTS = "detectron2://COCO-InstanceSegmentation/mask_rcnn_R_50_FPN_3x/137849600/model_final_f10217.pkl"
detector = DefaultPredictor(cfg)
# Norfair
video = Video(input_path="video.mp4")
tracker = Tracker(distance_function="euclidean", distance_threshold=20)
for frame in video:
detections = detector(cv2.cvtColor(frame, cv2.COLOR_BGR2RGB))
detections = [Detection(p) for p in detections['instances'].pred_boxes.get_centers().cpu().numpy()]
tracked_objects = tracker.update(detections=detections)
draw_tracked_objects(frame, tracked_objects)
video.write(frame)
```
The video and drawing tools use OpenCV frames, so they are compatible with most Python video code available online. The point tracking is based on [SORT](https://arxiv.org/abs/1602.00763) generalized to detections consisting of a dynamically changing number of points per detection.
## Motivation
Trying out the latest state-of-the-art detectors normally requires running repositories that weren't intended to be easy to use. These tend to be repositories associated with a research paper describing a novel new way of doing detection, and they are therefore intended to be run as a one-off evaluation script to get some result metric to publish on a particular research paper. This explains why they tend to not be easy to run as inference scripts, or why extracting the core model to use in another standalone script isn't always trivial.
Norfair was born out of the need to quickly add a simple layer of tracking over a wide range of newly released SOTA detectors. It was designed to seamlessly be plugged into a complex, highly coupled code base, with minimum effort. Norfair provides a series of modular but compatible tools, which you can pick and choose to use in your project.
## Comparison to other trackers
Norfair's contribution to Python's object tracker library repertoire is its ability to work with any object detector by being able to work with a variable number of points per detection, and the ability for the user to heavily customize the tracker by creating their own distance function.
If you are looking for a tracker, here are some other projects worth noting:
- [**OpenCV**](https://opencv.org) includes several tracking solutions like [KCF Tracker](https://docs.opencv.org/3.4/d2/dff/classcv_1_1TrackerKCF.html) and [MedianFlow Tracker](https://docs.opencv.org/3.4/d7/d86/classcv_1_1TrackerMedianFlow.html) which are run by making the user select a part of the frame to track, and then letting the tracker follow that area. They tend not to be run on top of a detector and are not very robust.
- [**dlib**](http://dlib.net) includes a correlation single object tracker. You have to create your own multiple object tracker on top of it yourself if you want to track multiple objects with it.
- [**AlphaPose**](https://github.com/MVIG-SJTU/AlphaPose) just released a new version of their human pose tracker. This tracker is tightly integrated into their code base, and to the task of tracking human poses.
- [**SORT**](https://github.com/abewley/sort) and [**Deep SORT**](https://github.com/nwojke/deep_sort) are similar to this repo in that they use Kalman filters (and a deep embedding for Deep SORT), but they are hardcoded to a fixed distance function and to tracking boxes. Norfair also adds some filtering when matching tracked objects with detections, and changes the Hungarian Algorithm for its own distance minimizer. Both these repos are also released under the GPL license, which might be an issue for some individuals or companies because the source code of derivative works needs to be published.
## Benchmarks
[MOT17](https://motchallenge.net/data/MOT17/) and [MOT20](https://motchallenge.net/data/MOT17/) results obtained using [motmetrics4norfair](https://github.com/tryolabs/norfair/tree/master/demos/motmetrics4norfair) demo script on the `train` split. We used detections obtained with [ByteTrack's](https://github.com/ifzhang/ByteTrack) YOLOX object detection model.
| MOT17 Train | IDF1 IDP IDR | Rcll | Prcn | MOTA MOTP |
| :---------: | :---------------: | :---: | :---: | :---------: |
| MOT17-02 | 61.3% 63.6% 59.0% | 86.8% | 93.5% | 79.9% 14.8% |
| MOT17-04 | 93.3% 93.6% 93.0% | 98.6% | 99.3% | 97.9% 07.9% |
| MOT17-05 | 77.8% 77.7% 77.8% | 85.9% | 85.8% | 71.2% 14.7% |
| MOT17-09 | 65.0% 67.4% 62.9% | 90.3% | 96.8% | 86.8% 12.2% |
| MOT17-10 | 70.2% 72.5% 68.1% | 87.3% | 93.0% | 80.1% 18.7% |
| MOT17-11 | 80.2% 80.5% 80.0% | 93.0% | 93.6% | 86.4% 11.3% |
| MOT17-13 | 79.0% 79.6% 78.4% | 90.6% | 92.0% | 82.4% 16.6% |
| OVERALL | 80.6% 81.8% 79.6% | 92.9% | 95.5% | 88.1% 11.9% |
| MOT20 Train | IDF1 IDP IDR | Rcll | Prcn | MOTA MOTP |
| :---------: | :---------------: | :---: | :---: | :---------: |
| MOT20-01 | 85.9% 88.1% 83.8% | 93.4% | 98.2% | 91.5% 12.6% |
| MOT20-02 | 72.8% 74.6% 71.0% | 93.2% | 97.9% | 91.0% 12.7% |
| MOT20-03 | 93.0% 94.1% 92.0% | 96.1% | 98.3% | 94.4% 13.7% |
| MOT20-05 | 87.9% 88.9% 87.0% | 96.0% | 98.1% | 94.1% 13.0% |
| OVERALL | 87.3% 88.4% 86.2% | 95.6% | 98.1% | 93.7% 13.2% |
## Commercial support
Tryolabs can provide commercial support, implement new features in Norfair or build video analytics tools for solving your challenging problems. Norfair powers several video analytics applications, such as the [face mask detection](https://tryolabs.com/blog/2020/07/09/face-mask-detection-in-street-camera-video-streams-using-ai-behind-the-curtain/) tool.
If you are interested, please [contact us](mailto:hello@tryolabs.com).
## Citing Norfair
For citations in academic publications, please export your desired citation format (BibTeX or other) from [Zenodo](https://doi.org/10.5281/zenodo.5146253).
## License
Copyright © 2022, [Tryolabs](https://tryolabs.com). Released under the [BSD 3-Clause](https://github.com/tryolabs/norfair/blob/master/LICENSE).
%prep
%autosetup -n norfair-2.2.0
%build
%py3_build
%install
%py3_install
install -d -m755 %{buildroot}/%{_pkgdocdir}
if [ -d doc ]; then cp -arf doc %{buildroot}/%{_pkgdocdir}; fi
if [ -d docs ]; then cp -arf docs %{buildroot}/%{_pkgdocdir}; fi
if [ -d example ]; then cp -arf example %{buildroot}/%{_pkgdocdir}; fi
if [ -d examples ]; then cp -arf examples %{buildroot}/%{_pkgdocdir}; fi
pushd %{buildroot}
if [ -d usr/lib ]; then
find usr/lib -type f -printf "/%h/%f\n" >> filelist.lst
fi
if [ -d usr/lib64 ]; then
find usr/lib64 -type f -printf "/%h/%f\n" >> filelist.lst
fi
if [ -d usr/bin ]; then
find usr/bin -type f -printf "/%h/%f\n" >> filelist.lst
fi
if [ -d usr/sbin ]; then
find usr/sbin -type f -printf "/%h/%f\n" >> filelist.lst
fi
touch doclist.lst
if [ -d usr/share/man ]; then
find usr/share/man -type f -printf "/%h/%f.gz\n" >> doclist.lst
fi
popd
mv %{buildroot}/filelist.lst .
mv %{buildroot}/doclist.lst .
%files -n python3-norfair -f filelist.lst
%dir %{python3_sitelib}/*
%files help -f doclist.lst
%{_docdir}/*
%changelog
* Tue May 30 2023 Python_Bot <Python_Bot@openeuler.org> - 2.2.0-1
- Package Spec generated
|